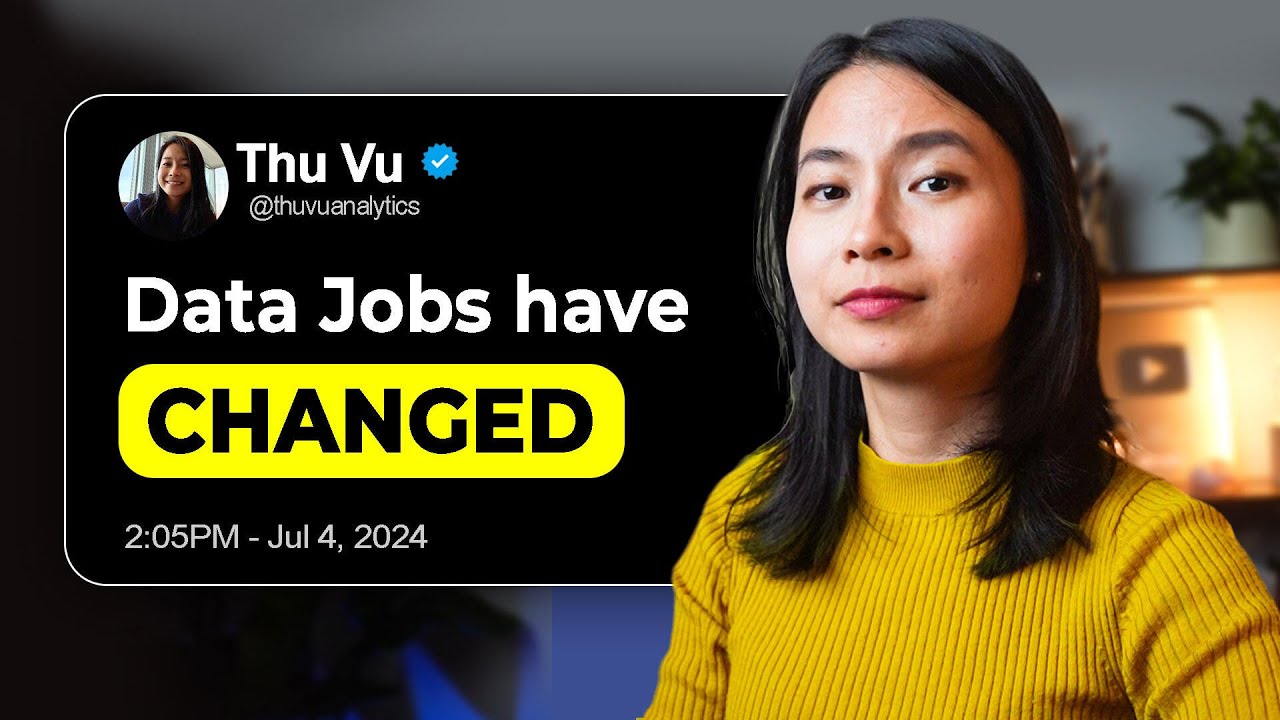
Create articles from any YouTube video or use our API to get YouTube transcriptions
Start for freeThe Current State of the Data Job Market
The data job market has undergone significant changes in recent years, prompting professionals to reassess their career prospects and adapt to new realities. Despite predictions of explosive growth for data science, statistics, and market research analyst roles by the U.S. Bureau of Labor Statistics, many individuals are finding it challenging to secure positions in the field. This article examines five key trends observed in the current data job market and provides insights into how professionals can position themselves for success.
Job Market Stability Amidst Tech Industry Turbulence
Contrary to expectations, the data job market has shown remarkable resilience in the face of widespread tech industry layoffs. Since the end of 2022, the number of job postings for data scientists, data analysts, and data engineers has decreased by approximately 15%. However, this figure has remained relatively stable since the beginning of 2023.
Interestingly, the data job market appears to be somewhat insulated from the broader tech industry trends. Despite two significant waves of layoffs in the tech sector since the COVID-19 pandemic—one at the beginning of 2023 and another at the start of 2024—job postings for data roles have not been significantly affected.
This stability can be observed across various job titles within the data field. While the market may be more competitive compared to the past two years, with companies becoming more selective in their hiring processes, opportunities continue to exist for skilled professionals.
Economic Factors Influencing Hiring Decisions
The current economic climate, characterized by uncertain growth and high interest rates, has led companies to tighten their budgets and prioritize efficiency and cost reduction. As a result, employers are seeking candidates with skills that align with these objectives. This shift in focus may contribute to the perception that landing a data job has become more challenging.
However, this trend should not discourage aspiring data professionals. Instead, it highlights the importance of strategically positioning oneself in the job market and developing skills that address the evolving needs of businesses.
Technical Skills in High Demand
As the data field continues to evolve, certain technical skills have emerged as clear frontrunners in terms of demand and relevance. Understanding these trends is crucial for professionals looking to enhance their marketability and stay competitive in the job market.
The Dominance of Python
Python has solidified its position as the primary programming language for data professionals. According to recent surveys, an overwhelming 86% of data scientists reported using Python as their main language for current projects. An additional 10% indicated that they use Python as a secondary language.
This widespread adoption of Python spans various aspects of data work, including:
- Data analysis
- Machine learning
- Web development
The versatility and extensive ecosystem of Python make it an invaluable tool for data professionals across different specializations. For those entering the field or looking to expand their skill set, focusing on Python is now more critical than ever.
The Enduring Importance of SQL
While Python has gained significant traction, SQL remains a fundamental skill for data professionals. Analysis of job posting data over the past two years reveals that SQL consistently appears in up to 60% of all data-related job listings, often alongside Python.
The persistent demand for SQL skills underscores its continued relevance in the data industry. SQL's importance extends beyond simple query writing; it encompasses a broader understanding of data management and manipulation.
Wes McKinney, the creator of the popular Python library Pandas, emphasizes the value of SQL proficiency:
"Learning SQL is actually a really good skill. It's not just learning SQL the language, but learning how to think about datasets, designing schemas, organizing data, relational algebra, and knowing how to use data execution engines, data warehouses, SQL engines, file formats, and the basics of data storage and partitioning."
McKinney argues that these skills enable professionals to be more effective users of various data tools and platforms, such as dbt, Snowflake, and Databricks.
Real-World Application of SQL Skills
The practical value of advanced SQL knowledge becomes evident in complex data projects. For instance, working with massive datasets, such as billions of bank transaction records for a money laundering risk classification model, requires a deep understanding of SQL optimization techniques.
Professionals who can effectively use partitioning, load balancing, and other advanced SQL concepts can significantly improve query performance and overall project efficiency. This level of expertise can set candidates apart in a competitive job market.
Emerging Roles in the Data Field
As the data industry continues to evolve, new roles are emerging to meet the changing needs of businesses. One of the most notable developments in recent years has been the rise of AI Engineers.
The Rise of AI Engineers
The rapid advancements in large language models (LLMs) over the past two years have given birth to a new and exciting role: the AI Engineer. This position differs from traditional data science and machine learning roles in several key aspects:
-
Educational requirements: Unlike many data science positions that often require a Ph.D., AI Engineer roles typically do not have such stringent academic prerequisites.
-
Skill focus: AI Engineers need in-depth knowledge of LLMs, prompt engineering, and AI agent workflow engineering.
-
Application-oriented: These professionals tend to work more on the product and user end of the AI spectrum, focusing on practical applications of AI technologies.
AI Engineers are responsible for developing applications that leverage pre-trained AI models or foundation models to address specific business challenges. Their work often involves:
- Implementing AI models
- Conducting prompt engineering
- Fine-tuning models for specific use cases
- Tailoring AI workflows to business needs
- Evaluating and optimizing AI applications
Growth in AI Engineer Positions
The demand for AI Engineers has been growing at a remarkable rate. According to job posting data, AI Engineer positions have been increasing much faster than traditional machine learning engineer roles. In fact, AI Engineer job listings surpassed those for machine learning engineers in May 2023.
This trend is likely to continue as more businesses seek to incorporate AI capabilities into their operations. The recent partnership between PwC and OpenAI, which aims to scale AI capabilities across businesses, is just one example of the growing demand for AI expertise in various industries.
Transitioning to an AI Engineer Role
For data professionals interested in transitioning to an AI Engineer role, focusing on the following areas can be beneficial:
- Mathematical foundations
- Basic statistics
- Python programming
- Machine learning fundamentals
- Deep learning principles
Additional resources for skill development include:
- Machine Learning Specialization (Coursera)
- Deep Learning Specialization (Coursera)
- Fast.ai Deep Learning courses
After building a strong foundation, professionals can specialize in specific areas of AI, such as:
- Natural Language Processing (NLP)
- Computer Vision
- Reinforcement Learning
Emerging Quality Assurance Roles in AI
As AI applications become more prevalent, new roles are emerging to ensure their quality and performance. These positions, often referred to as Quality Assurance Business Analysts or QA Analysts, focus on:
- Investigating LLM outputs
- Designing A/B tests for AI applications
- Creating dashboards to monitor AI performance
While these roles are not yet widely reflected in job posting data, they represent an emerging opportunity for professionals with strong analytical skills and a background in data science.
The Rise of Freelancing in the Data World
The data industry is witnessing a significant shift towards freelance and contract work. This trend offers new opportunities for professionals seeking flexibility and diverse work experiences.
Increasing Demand for Freelancers
In 2024, there has been a notable increase in job postings seeking contractors and freelancers in the data field. This trend is particularly exciting for professionals interested in:
- Part-time work
- Flexible job arrangements
- Rapid skill development
- Building a diverse project portfolio
Freelancing allows data professionals to work on various business problems across different domains, exposing them to a wide range of data types and analytical tools.
Finding Freelance Opportunities
For those new to freelancing, finding the first client can be challenging. Here are some strategies to get started:
-
Leverage your network: In the U.S., most freelancers find work through previous clients, friends, family, and professional contacts.
-
Utilize social media: Share relevant projects on platforms like LinkedIn to showcase your skills and indicate your availability for freelance work.
-
Start local: Reach out to small businesses in your area or friends and family members who might need data-related assistance.
-
Build a portfolio: Start with small projects to gain experience and build a diverse portfolio of work.
-
Explore online platforms: Once you have some experience, consider using platforms like Upwork and Fiverr to find additional opportunities.
The Impact of Low-Code and No-Code Tools
The rise of low-code and no-code development platforms is transforming the data analytics landscape, making advanced analysis more accessible to a broader range of professionals.
Democratizing Data Analytics
Low-code and no-code tools, often powered by AI, are enabling individuals without traditional coding or data science backgrounds to perform complex data tasks. These platforms provide simplified interfaces that allow users to:
- Prepare and clean data
- Conduct analyses
- Create data visualizations
- Build basic machine learning models
The average forecasted low-code market size is expected to grow by approximately 23% annually from 2023 to 2030, indicating the increasing adoption of these tools across industries.
Implications for Data Professionals
The proliferation of low-code and no-code tools has several implications for the data job market:
-
Automation of entry-level tasks: Many basic data analysis tasks are being automated, potentially impacting job opportunities for those just entering the field.
-
Opportunities for non-technical experts: Professionals with domain expertise but limited technical skills can now contribute more directly to data analysis projects.
-
Specialization of roles: As basic tasks become automated, data professionals may need to focus on more specialized and complex aspects of data work.
-
Emphasis on domain knowledge: Understanding specific business domains and their unique data challenges may become increasingly valuable.
Adapting to the Changing Landscape
To thrive in this evolving environment, data professionals should consider:
-
Broadening their job search: Look beyond traditional data analyst or data scientist titles to roles that combine data skills with specific domain knowledge.
-
Developing specialized skills: Focus on areas that are less likely to be automated, such as advanced statistical analysis or machine learning model development.
-
Enhancing soft skills: Improve communication, problem-solving, and business acumen to provide value beyond technical data manipulation.
Strategies for Success in the Evolving Data Job Market
As the data job market continues to evolve, professionals must adapt their strategies to remain competitive and successful. Here are key approaches to consider:
1. Focus on Excellence
Regardless of market conditions, companies always value individuals who excel in their field. Strive to be exceptional at what you do by:
- Continuously updating your skills
- Staying informed about industry trends
- Seeking opportunities to apply your knowledge in practical settings
2. Build a Strong Portfolio
Demonstrate your skills and experience through a robust portfolio of projects. This can include:
- Personal projects that showcase your creativity and problem-solving abilities
- Volunteer work for non-profit organizations or open-source projects
- Freelance projects that highlight your ability to deliver results in real-world scenarios
A diverse and impressive portfolio can set you apart from other candidates and provide tangible evidence of your capabilities.
3. Develop Your Network
Networking remains a crucial aspect of career development in the data field. Effective networking strategies include:
- Attending industry conferences and meetups
- Participating in online forums and communities
- Engaging with professionals on social media platforms like LinkedIn
- Contributing to open-source projects or industry publications
Building a strong professional network can lead to job opportunities, collaborations, and valuable insights into industry trends.
4. Embrace Continuous Learning
The rapid pace of change in the data field necessitates a commitment to lifelong learning. Stay ahead of the curve by:
- Enrolling in online courses or bootcamps to learn new skills
- Pursuing relevant certifications in your area of specialization
- Reading industry publications and research papers
- Experimenting with new tools and technologies in your personal projects
5. Develop Cross-Functional Skills
As data becomes increasingly integral to various business functions, developing skills that bridge data analysis with other domains can be highly valuable. Consider developing expertise in areas such as:
- Business analytics
- Marketing analytics
- Financial modeling
- Supply chain optimization
- Healthcare informatics
6. Cultivate Soft Skills
While technical skills are crucial, soft skills can often be the differentiating factor in career advancement. Focus on developing:
- Communication skills: Ability to explain complex concepts to non-technical stakeholders
- Leadership: Capacity to guide teams and projects effectively
- Problem-solving: Aptitude for tackling complex, ambiguous challenges
- Adaptability: Willingness to learn and adjust to new situations quickly
7. Stay Informed About Industry Trends
Keep abreast of the latest developments in the data field by:
- Following industry thought leaders on social media
- Subscribing to relevant newsletters and podcasts
- Participating in webinars and online events
- Joining professional associations related to data science and analytics
8. Consider Specialization
As the field becomes more competitive, specializing in a particular area of data science can help you stand out. Some areas to consider include:
- Natural Language Processing
- Computer Vision
- Time Series Analysis
- Geospatial Data Analysis
- Ethical AI and Responsible Data Science
9. Develop Business Acumen
Understanding how data insights translate into business value is increasingly important. Enhance your business knowledge by:
- Studying business case studies
- Learning about different business models and strategies
- Understanding key performance indicators (KPIs) in various industries
- Collaborating with business stakeholders on cross-functional projects
10. Be Proactive in Your Job Search
Take an active approach to your job search by:
- Customizing your resume and cover letter for each application
- Reaching out directly to companies you're interested in, even if they don't have current openings
- Leveraging your network for referrals and insider information about job opportunities
- Preparing thoroughly for interviews, including practicing common data science interview questions
Conclusion
The data job market is undoubtedly evolving, presenting both challenges and opportunities for professionals in the field. While certain aspects of data work are becoming more accessible through low-code and no-code tools, the demand for specialized skills and domain expertise continues to grow.
Key trends shaping the future of data jobs include:
- The continued dominance of Python and SQL as essential technical skills
- The emergence of AI Engineer roles and the growing importance of AI-related skills
- Increasing opportunities in freelance and contract work
- The impact of low-code and no-code tools on entry-level data tasks
- The growing emphasis on specialized knowledge and domain expertise
To thrive in this changing landscape, data professionals must remain adaptable, continuously update their skills, and focus on delivering tangible value to businesses. By staying informed about industry trends, building a strong portfolio, and cultivating a robust professional network, individuals can position themselves for success in the evolving data job market.
Remember, the key to long-term success in the data field lies in a combination of technical proficiency, business acumen, and the ability to communicate insights effectively. By focusing on these areas and remaining open to new opportunities and challenges, data professionals can navigate the changing job market with confidence and build rewarding, impactful careers.
Article created from: https://www.youtube.com/watch?v=iuO2D5nz_AE