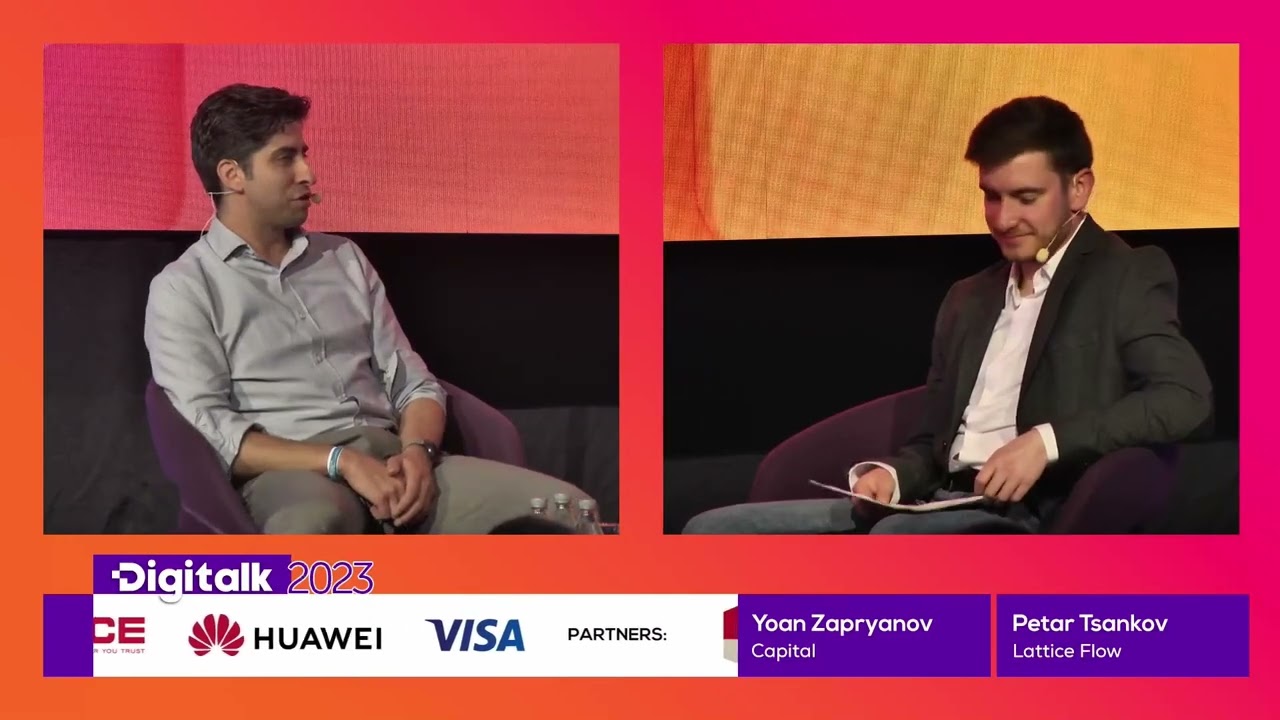
Create articles from any YouTube video or use our API to get YouTube transcriptions
Start for freeThe Current State of AI: Revolution Without Breakthrough
The past year has witnessed a significant shift in the field of artificial intelligence (AI), marking what many consider the beginning of an AI revolution. However, it's crucial to understand that this revolution isn't driven by new technological breakthroughs, but rather by the innovative application of existing technologies.
The Foundation of Modern AI
The core technologies powering today's AI advancements have been in development for years:
- Advanced hardware capabilities
- Neural network architectures, particularly the Transformer architecture
- Large language models trained on vast amounts of data
What changed in the past year was not the fundamental technology, but its application and accessibility.
ChatGPT: A Leap in Usability
OpenAI's ChatGPT model represents a significant milestone in AI usability. While not a technological breakthrough in itself, ChatGPT demonstrated:
- Improved alignment with human expectations
- Enhanced ability to provide relevant and coherent responses
- Unprecedented user adoption, with one billion users in just a few weeks
This rapid adoption underscores that the current AI revolution is not merely hype, but a genuine shift in how people interact with and utilize AI technologies.
The Evolution of AI: From Turing to Today
To fully appreciate the current state of AI, it's essential to understand its historical context:
- 1950s: Alan Turing, the father of computer science, defines the Turing test
- 1980s-1990s: AI experiences two "winters" of reduced funding and interest
- Present day: Data-driven systems and neural networks dominate the field
The current AI revolution builds upon decades of research and development, representing a culmination of efforts rather than a sudden emergence of new technologies.
Expert Models: The Future of AI in Business
While general-purpose AI models like ChatGPT have captured public attention, the future of AI in business lies in specialized "expert models."
Limitations of General-Purpose AI
General AI models, while impressive, have significant limitations:
- Lack of expertise in specific domains
- Inability to make critical decisions in specialized fields (e.g., medical diagnosis, financial investments)
The Rise of Expert Models
Business needs are driving the development of expert models:
- Trained on high-quality, domain-specific data
- Designed to solve particular problems with high accuracy
- Focused on delivering tangible business value
Challenges in Developing Expert Models
Creating effective expert models presents several challenges:
- Data curation and quality control
- Addressing biases in training data
- Developing data-efficient training methods
- Ensuring model reliability and consistency
These challenges cannot be solved simply by increasing data volume or computational power. They require fundamental advancements in AI research and development.
Short-Term vs. Long-Term AI Challenges
The AI industry faces both immediate and future challenges as it continues to evolve.
Short-Term Focus
In the near term, efforts are concentrated on:
- Rapidly developing and deploying specialized AI models
- Improving data quality and curation processes
- Enhancing model training efficiency
Long-Term Considerations
Looking ahead, the AI community must address:
- Fairness and bias in AI systems
- Security and robustness of AI models
- Ethical considerations in AI development and deployment
The Quest for Artificial General Intelligence (AGI)
As AI continues to advance, questions about the possibility of achieving Artificial General Intelligence (AGI) persist.
Defining and Measuring AGI
One of the primary challenges in discussing AGI is the lack of a clear, universally accepted definition or test:
- The Turing Test, while historically significant, is not a definitive measure of AGI
- There is no binary test to determine if a system has achieved AGI
Current Limitations
Despite rapid progress, current AI systems face significant hurdles in achieving AGI:
- Lack of logical reasoning capabilities comparable to humans
- Inability to effectively incorporate background knowledge
- Difficulty in generalizing learning from one domain to another
The Path Forward
Achieving AGI will likely require:
- Fundamental advances in AI training techniques
- New approaches to model architecture and design
- Incremental improvements in generalizability and adaptability
It's important to note that progress towards AGI may be gradual and difficult to pinpoint, rather than a sudden breakthrough.
Anthropomorphism and AI Perception
As AI systems become more advanced, questions arise about their potential for emotional understanding and human-like qualities.
Emotional AI: A Complex Question
The concept of AI with emotions or human-like consciousness remains highly speculative:
- Current AI models lack true understanding or emotional capacity
- Anthropomorphizing AI can lead to misunderstandings about its capabilities
Focus on Functionality
Instead of debating whether AI can have emotions, it's more productive to focus on:
- Improving AI's ability to solve specific problems
- Enhancing AI's usefulness and reliability in various applications
The Role of Benchmarks in AI Progress
While definitive tests for AGI may not exist, benchmarks play a crucial role in driving AI advancement:
- Datasets like ImageNet have been instrumental in computer vision progress
- Benchmarks provide measurable goals for researchers and developers
- Continuous improvement of benchmarks helps push the boundaries of AI capabilities
AI Regulation: Balancing Innovation and Safety
As AI becomes more prevalent and powerful, the question of regulation becomes increasingly important.
The Case for Regulation
Regulation of AI is necessary for several reasons:
- To prevent misuse of AI in potentially harmful applications (e.g., social scoring systems)
- To establish ethical guidelines for AI development and deployment
- To ensure public safety and trust in AI technologies
Challenges in AI Regulation
Effective AI regulation faces several challenges:
- Balancing innovation with safety concerns
- Avoiding overly restrictive policies that could hinder progress
- Keeping pace with rapidly evolving AI technologies
Regional Differences in Approach
Different regions are taking varied approaches to AI regulation:
- Europe tends to be more conservative and heavily regulated
- The United States often favors a more market-driven approach
These differences can lead to disparities in AI adoption and development across regions.
Finding the Right Balance
Effective AI regulation should:
- Prohibit clearly harmful applications of AI
- Allow market forces to guide development in beneficial areas
- Remain flexible enough to adapt to rapid technological changes
The Debate on Pausing AI Development
Recent proposals to pause AI development have sparked controversy in the tech community.
Arguments Against Pausing AI Development
Many experts argue against pausing AI development for several reasons:
- It's practically impossible to enforce a global pause on AI research
- Pausing public development could shift progress behind closed doors
- It could hinder beneficial advancements in AI technology
Alternative Approaches
Instead of pausing development, experts suggest focusing on:
- Increasing transparency in AI research and development
- Enhancing safety measures and ethical guidelines
- Promoting open collaboration in addressing AI challenges
The Future of AI: Optimism and Caution
Looking ahead, the future of AI presents both exciting possibilities and potential concerns.
Reasons for Optimism
- Rapid advancements in AI capabilities
- Potential for solving complex global challenges
- Continuous improvement in AI reliability and usefulness
Areas of Caution
- Unprecedented speed of AI development
- Potential short-term risks and disruptions
- Need for ongoing ethical considerations
Embracing Progress
Despite concerns, many experts advocate for continued AI development:
- Technological progress is a fundamental aspect of human evolution
- Focusing on responsible development rather than halting progress
- Addressing challenges through research and collaboration
AI on the Global Stage
The development of AI technology is not evenly distributed across the globe, with some regions leading while others lag behind.
Defining AI Leadership
Being "on the AI map" involves more than just using AI technology:
- Driving fundamental progress in AI research
- Publishing in top-tier AI conferences and journals
- Contributing to solving open challenges in AI security and reliability
Challenges for Emerging Tech Hubs
Regions like Eastern Europe face several challenges in becoming AI leaders:
- Lack of presence at major AI conferences
- Limited resources for fundamental AI research
- Brain drain of talented researchers to established tech hubs
Bridging the Gap
Efforts to elevate emerging regions in the AI field include:
- Establishing research centers to attract and retain top talent
- Fostering collaboration between local institutions and global AI leaders
- Investing in education and infrastructure to support AI development
Conclusion: Navigating the AI Revolution
As we continue to navigate the AI revolution, it's clear that we are witnessing a transformative period in technology. While not driven by singular breakthroughs, the current AI landscape is characterized by the innovative application of existing technologies, leading to unprecedented levels of adoption and integration into our daily lives.
The future of AI lies not just in general-purpose models, but in the development of specialized expert models that can deliver tangible value across various industries. This path forward is not without challenges, from addressing biases and ensuring data quality to grappling with complex ethical and regulatory issues.
As the field progresses, the focus should remain on responsible development, transparent research, and collaborative problem-solving. By embracing the potential of AI while thoughtfully addressing its challenges, we can work towards a future where AI technology enhances human capabilities and contributes positively to society.
The journey towards more advanced AI, including the potential development of AGI, will likely be gradual and marked by incremental improvements rather than sudden leaps. This evolutionary process will require ongoing dedication to research, ethical considerations, and adaptive regulatory frameworks.
Ultimately, the AI revolution is not just about technology—it's about how we as a society choose to shape and utilize these powerful tools. By maintaining a balance of optimism and caution, we can strive to harness the benefits of AI while mitigating potential risks, ensuring that the future of AI is one that aligns with human values and aspirations.
Article created from: https://www.youtube.com/watch?v=ow1N_W4Xc20&feature=youtu.be