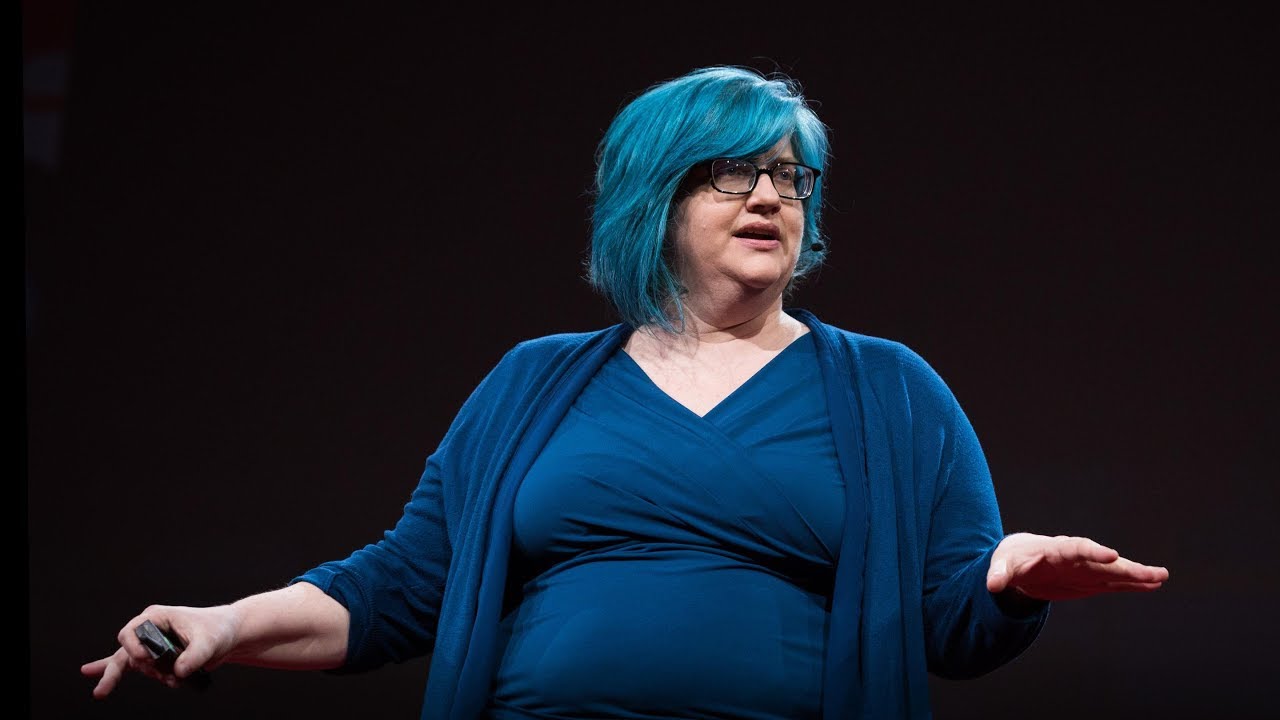
Create articles from any YouTube video or use our API to get YouTube transcriptions
Start for freeIn today's digital age, algorithms play a pivotal role in decision-making processes, from job applications to legal sentencing. However, beneath the surface of these seemingly neutral algorithms lies a complex web of biases that can have profound impacts on individuals and society. This article delves into the hidden dangers of algorithms and underscores the urgency of demanding accountability for these digital decision-makers.
The Illusion of Objectivity in Algorithms
Algorithms are often perceived as objective and scientific, a belief that developers and companies eagerly propagate. Yet, at their core, algorithms are opinions embedded in code, reflecting the biases and values of those who create them. For instance, a simple daily task like preparing a meal can be viewed through the lens of algorithmic decision-making, where the ingredients available, time, and personal definitions of success (such as ensuring kids eat vegetables) shape the outcome. This analogy beautifully illustrates how even the most mundane decisions are influenced by personal biases, challenging the notion of algorithms as impartial arbiters.
The Real-World Consequences of Algorithmic Bias
The impact of biased algorithms extends far beyond the kitchen. In educational settings, algorithms like the "value-added model" have been used to score teachers, leading to unjust outcomes based on flawed and opaque criteria. These algorithmic assessments can result in the shaming and even firing of dedicated educators based on unreliable data. Similarly, in the criminal justice system, algorithms tasked with predicting recidivism risk have shown racial bias, assigning higher risk scores to black individuals compared to their white counterparts, despite similar backgrounds. These examples highlight how algorithms, under the guise of objectivity, can perpetuate and amplify societal inequalities.
The Dangerous Myth of Algorithmic Fairness
One might hope that machine learning and data-driven approaches could eliminate human bias from decision-making processes. However, algorithms trained on historical data inevitably learn and replicate the biases present in that data. For example, an algorithm designed to streamline hiring processes at a company with a history of gender discrimination would likely continue to disadvantage women. This phenomenon, where algorithms automate and perpetuate the status quo, underscores the fallacy of algorithmic fairness. Without critical examination and intervention, algorithms will continue to encode and reinforce existing biases.
Demanding Accountability and Transparency
The pervasive influence of algorithms in our lives necessitates a new approach to their development and deployment. Rather than accepting algorithmic decisions as infallible, we must recognize them as constructs that reflect human prejudices. This realization opens the door to scrutinizing and improving algorithms through practices like algorithmic audits, which assess data integrity, definitions of success, accuracy, and the potential for long-term negative feedback loops. By holding algorithms to account, we can begin to mitigate their harmful impacts and work towards more equitable outcomes.
A Call to Action
The era of uncritical acceptance of algorithms must come to an end. Data scientists have a responsibility to act as translators of ethical discussions, ensuring that algorithms serve the public good rather than perpetuate harm. Meanwhile, the broader public must engage in a political fight for transparency and accountability from those who deploy algorithms. Together, we can challenge the unchecked power of algorithms and strive for a future where technology serves to uplift rather than oppress.
In conclusion, it's crucial for society to recognize the inherent biases within algorithms and demand greater accountability from those who create and deploy them. By doing so, we can prevent these digital decision-makers from reinforcing societal inequalities and work towards a more just and fair world.
For a deeper understanding of the complexities and implications of algorithmic bias, watch the full discussion here: Original Video.