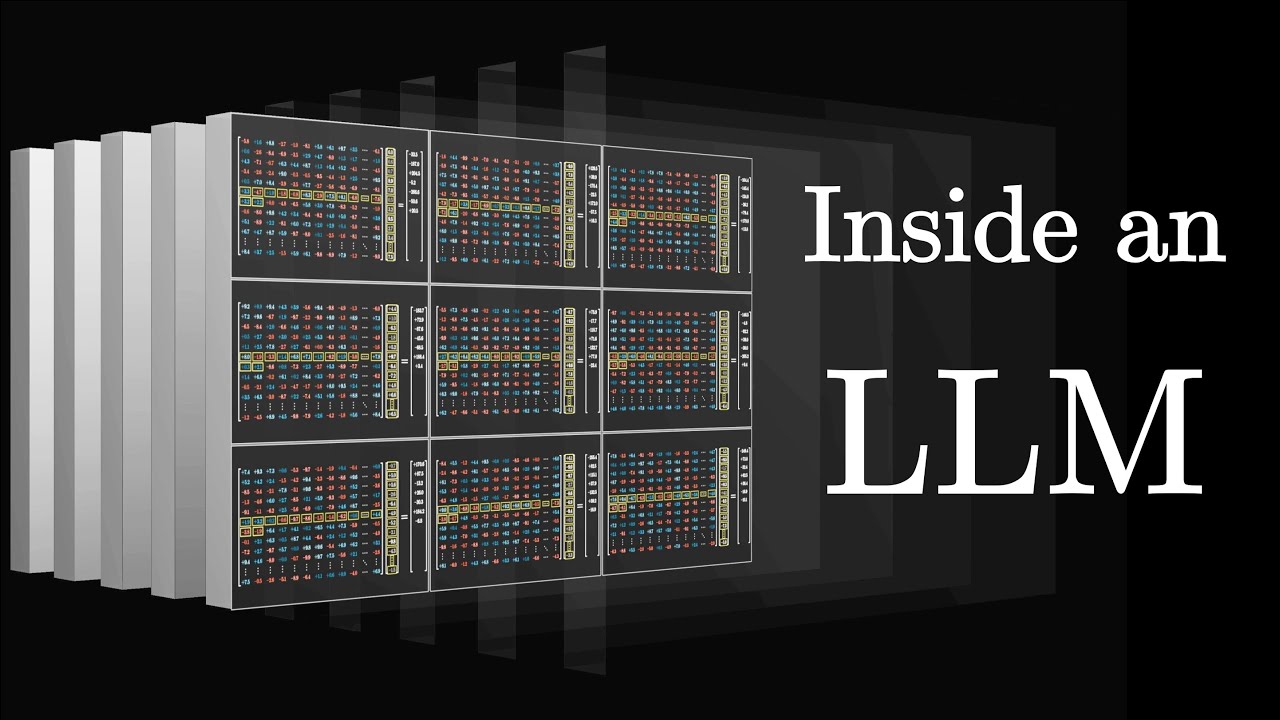
Create articles from any YouTube video or use our API to get YouTube transcriptions
Start for freeUnderstanding Generative Pretrained Transformers (GPT)
The term GPT stands for Generative Pretrained Transformer. This breakthrough technology has significantly contributed to the current surge in AI advancements, making it possible to generate text, transcribe audio into text, and even convert simple text descriptions into images. At its core, GPT is a type of neural network known as a transformer, which is pretrained on vast datasets, allowing it to generate new content based on the input it receives.
The Magic Behind GPT
The real magic of GPT lies in its architecture, particularly the transformer model. Transformers employ a unique mechanism called attention, allowing the model to focus on different parts of the input data, making sense of how words or tokens relate to each other in varying contexts. This attention mechanism is crucial for understanding the nuances of language, whether it's for translating languages, generating coherent text, or interpreting the meaning behind a text snippet.
How GPT Generates Text
To generate text, GPT follows a simple yet ingenious process. It begins with a seed text and predicts what comes next, creating a probability distribution over possible subsequent chunks of text. By sampling from this distribution and adding the new text to the original seed, GPT can generate lengthy and coherent pieces of text, one piece at a time. This capability is not just theoretical; it's the underlying process for AI tools like ChatGPT, which can engage in conversations, write stories, or even generate creative content based on textual prompts.
The Role of Transformers in AI
Transformers, the backbone of GPT, have revolutionized the field of AI. Introduced by Google in 2017, their initial purpose was for language translation. However, their flexibility has allowed for a wide array of applications, from speech synthesis to creating images from text descriptions with tools like Dolly and Midjourney. The ability of transformers to handle and interpret vast amounts of data accurately has been a game-changer, making AI more versatile and powerful than ever before.
Breaking Down the Transformer Model
At a high level, the transformer model processes data in steps. First, the input is divided into tokens, which could be words, parts of words, or even patches of an image. Each token is then represented as a vector in a high-dimensional space, where similar tokens have vectors that are close to each other. This representation allows the model to understand the meaning of each token in context. Through layers of attention blocks and multi-layer perceptrons, the tokens interact, updating their meanings based on the surrounding context. Finally, the model predicts the next token by creating a probability distribution over all possible tokens, effectively generating new content.
The Future of AI With GPT
The development and refinement of GPT models, such as GPT-3, have demonstrated the vast potential of AI. With billions of parameters, these models can generate text that is not only coherent but also contextually relevant, opening up new possibilities for AI applications. From writing assistance and customer service bots to more creative pursuits like story generation and graphic design, GPT is at the forefront of AI innovation.
The journey into understanding and leveraging the power of GPT and transformers is just beginning. As we delve deeper into how these models work and find new ways to improve and apply them, the future of AI looks brighter and more promising than ever.
For a more detailed exploration of how transformers and GPT models work, including their applications and impact on the field of AI, watch the full video here.