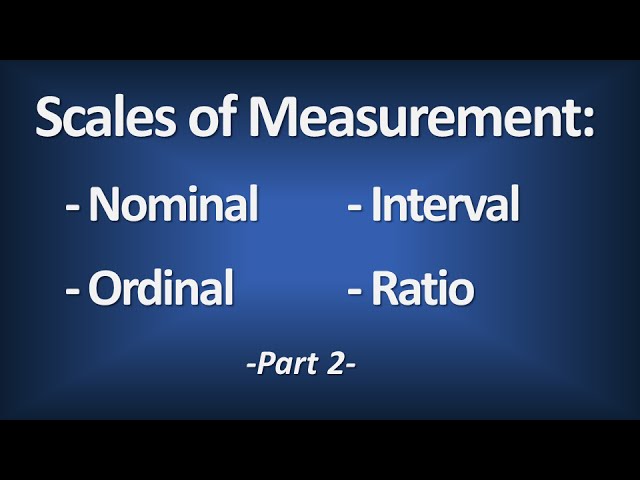
Create articles from any YouTube video or use our API to get YouTube transcriptions
Start for freeIn the realm of data analysis, understanding the scales of measurement is crucial for interpreting and utilizing data correctly. These scales - nominal, ordinal, interval, and ratio - provide a foundation for statistical analysis, each with its unique characteristics and applications. Let's delve into what makes each scale distinct and how they compare with one another, shedding light on their practical implications in data handling and interpretation.
Nominal Scale
The nominal scale categorizes data without implying any order. It is essentially used for labeling variables, without any quantitative value. For example, gender, nationality, and eye color are all categorized using the nominal scale. It's important to note that, with nominal data, one cannot perform arithmetic operations because it merely distinguishes between items or subjects based on names or categories.
Ordinal Scale
Moving up the complexity ladder, the ordinal scale not only categorizes data but also introduces an order among the categories. However, this scale does not quantify the difference between these categories. A classic example of the ordinal scale in action is the finishing positions in a race (first, second, third, etc.). While we can clearly identify an order, the specific differences in performance or time between these positions are not defined by the scale itself.
Interval Scale
The interval scale offers more sophistication by not only categorizing and ordering data but also quantifying the difference between each category. However, it lacks a true zero point, meaning the absence of a quantity is not defined. Temperature is a typical example used to explain the interval scale. Here, differences between temperatures are meaningful, and we can say how much warmer or colder one day is compared to another. Still, the scale does not define the absence of temperature; thus, zero degrees does not mean 'no temperature'.
Ratio Scale
The ratio scale encompasses all the characteristics of the interval scale and adds a true zero point, making ratios meaningful. Weight is a prime example of the ratio scale at work. Not only can we measure differences and establish a meaningful order, but we also can say that zero pounds signify the absence of weight, and ten pounds is twice as much as five pounds. This scale provides the most information and allows for the broadest range of mathematical operations.
Comparing the Scales
Interval vs. Ordinal
When comparing interval and ordinal scales, consider the difference in the level of detail each provides. For instance, temperature (interval) gives us precise measurements with equal differences, while race positions (ordinal) tell us the order without specifying the exact differences in performance.
Interval vs. Ratio
The key distinction between interval and ratio scales lies in the presence of a true zero point in the latter, making ratios (e.g., weights) meaningful. In contrast, temperatures (interval) do not offer a true zero point where no temperature exists, and their ratios are not meaningful in the same way.
Nominal vs. Other Scales
Nominal data, such as baseball uniform numbers, differ significantly from the other scales as they only serve to identify or categorize without implying any order, measurable difference, or ratio. The concept of zero in nominal data does not indicate absence, unlike in the ratio scale.
Understanding these scales of measurement is fundamental for anyone engaged in data analysis, research, or statistics. Each scale serves its purpose and applies to various types of data, enabling researchers and analysts to make informed decisions on how to process and interpret their data effectively.
For a deeper dive into scales of measurement and their implications, watch the comprehensive explanation here.