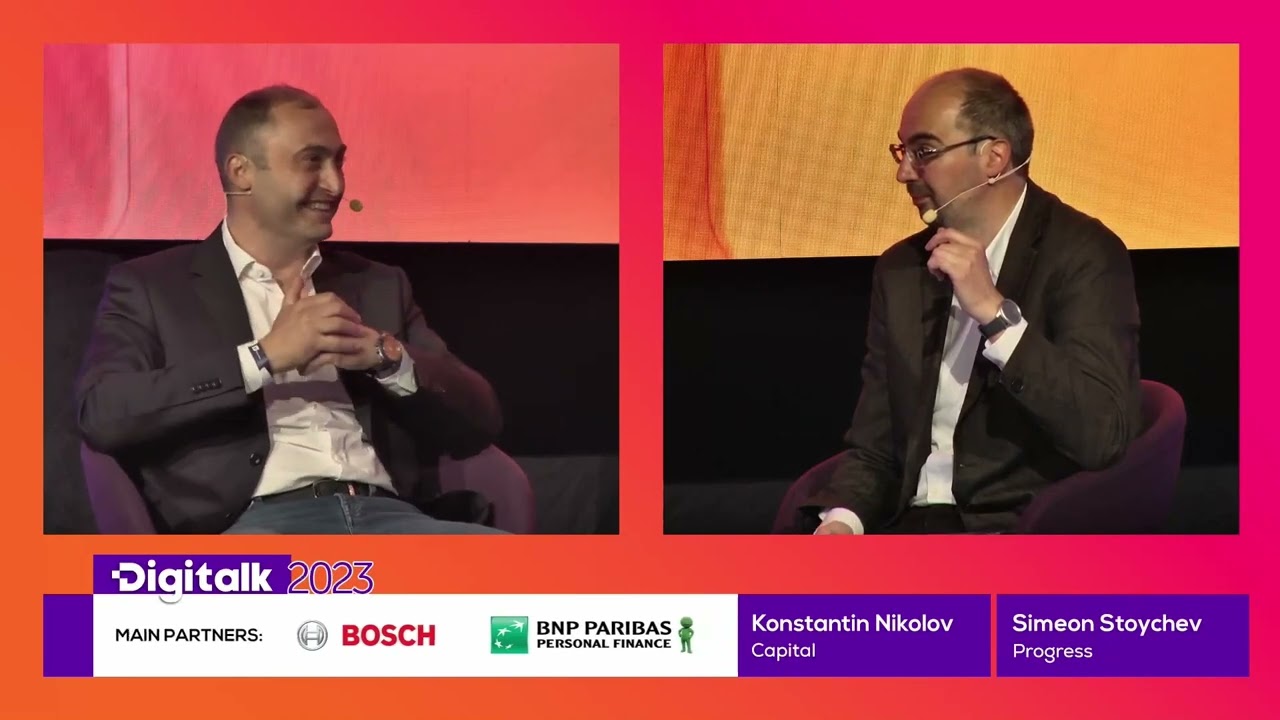
Create articles from any YouTube video or use our API to get YouTube transcriptions
Start for freeThe Hidden Risks of AI: Unveiling Data Bias
Artificial Intelligence (AI) has become an integral part of modern business operations, promising improved efficiency and decision-making. However, a recent global study by Progress Software has shed light on a critical issue that many organizations are overlooking: data bias in AI systems. This comprehensive article delves into the findings of the study, explores the implications of data bias, and offers insights into how businesses can address this growing concern.
Understanding Data Bias in AI
Before we dive into the study's findings, it's crucial to understand what data bias means in the context of AI:
- Data bias refers to systematic errors in data collection or analysis that can lead to inaccurate or unfair outcomes.
- In machine learning, bias often occurs when algorithms are trained on biased datasets, perpetuating and amplifying existing prejudices.
- The consequences of biased AI systems can be far-reaching, potentially leading to discrimination against individuals or groups in real-world scenarios.
Progress Software's Global Study: Key Findings
Progress Software conducted an extensive survey of 640 large organizations worldwide, each with over 500 employees and already utilizing some form of AI. The study, titled "The Hidden Risk of AI," aimed to assess the awareness and preparedness of businesses regarding AI data bias. Here are the key findings:
Awareness vs. Action
- 78% of businesses and decision-makers believe that data bias will become a significant concern.
- Only 13% of organizations are actively taking steps to address the issue.
This stark contrast highlights a critical gap between recognizing the problem and implementing solutions.
Prevalence of Data Bias
- 65% of surveyed organizations believe that data bias already exists within their AI systems.
This statistic is particularly alarming, as it suggests that biased decision-making may already be impacting various aspects of these businesses.
Global vs. Bulgarian Perspectives
The study also provided specific insights into the Bulgarian market, revealing some interesting contrasts:
- 95% of Bulgarian respondents (compared to 66% globally) anticipate becoming more reliant on AI and machine learning for decision-making.
- 79% of Bulgarian organizations (compared to 65% globally) believe that data bias already exists in their systems.
These figures suggest that while Bulgarian businesses are more enthusiastic about AI adoption, they are also more aware of the potential risks associated with data bias.
The Implications of Data Bias
The prevalence of data bias in AI systems raises several concerns:
Perpetuating Discrimination
Biased AI algorithms can reinforce existing societal prejudices, leading to unfair treatment of certain groups in areas such as:
- Hiring and recruitment
- Loan approvals
- Criminal justice decisions
- Healthcare resource allocation
Flawed Decision-Making
Organizations relying on biased AI systems may make suboptimal business decisions, potentially impacting:
- Customer segmentation and targeting
- Product development
- Resource allocation
- Risk assessment
Reputational Risks
Companies found to be using biased AI systems may face:
- Public backlash
- Legal challenges
- Damage to brand reputation
- Loss of customer trust
Strategies for Addressing Data Bias
Given the significant risks associated with data bias, it's crucial for organizations to take proactive steps to address this issue. Here are some strategies recommended by experts:
Implement Robust Data Governance
- Establish clear policies and procedures for data collection, storage, and usage.
- Regularly audit data sources and AI models for potential biases.
- Implement data quality checks and validation processes.
Enhance Data Context with Metadata
- Provide additional context to data points through comprehensive metadata.
- Use tools like MarkLogic (recently acquired by Progress Software) to manage complex data and metadata at scale.
Utilize Data Classification Tools
- Employ automated tools to categorize and flag potentially biased data.
- Implement systems that can analyze data at scale and identify patterns indicative of bias.
Incorporate Human Oversight
- Establish processes for human review of AI decisions, especially in high-stakes scenarios.
- Create diverse teams to review and validate AI models and outputs.
Invest in Education and Training
- Provide comprehensive training on data bias and ethical AI practices to all relevant staff.
- Foster a culture of awareness and responsibility around AI implementation.
Leverage Advanced AI Techniques
- Explore techniques such as federated learning and differential privacy to reduce bias in AI models.
- Implement fairness constraints and bias mitigation algorithms in machine learning pipelines.
The Role of Regulation in Addressing AI Bias
As the awareness of AI bias grows, so does the call for regulatory oversight. The European Union has recently made significant strides in this area:
The EU AI Act
- Recently approved at the committee level
- Expected to receive final approval in June
- Aims to set ethical guidelines and establish social control over AI technology
While regulation is a step in the right direction, it faces several challenges:
- Keeping pace with rapidly evolving technology
- Balancing innovation with control
- Ensuring global consistency in AI governance
The Human Factor in AI Development
Despite the advanced capabilities of AI, the human element remains crucial in ensuring responsible and ethical AI implementation:
Combining Human Intelligence with AI
- Leveraging human expertise to guide AI development and decision-making
- Using AI as a tool to augment human capabilities rather than replace them
The Importance of Diverse Teams
- Ensuring that AI development teams represent diverse perspectives and backgrounds
- Reducing the risk of inadvertent bias being introduced into AI systems
Continuous Learning and Adaptation
- Recognizing that AI systems require ongoing monitoring and refinement
- Fostering a culture of continuous improvement and ethical consideration
Case Studies: AI Bias in Action
To better understand the real-world implications of AI bias, let's examine a few notable cases:
Recruitment AI Bias
A major tech company discovered that its AI-powered recruitment tool was biased against women due to historical hiring data.
Lesson learned: Historical data may perpetuate existing biases, requiring careful curation and balancing of training datasets.
Facial Recognition Failures
Several facial recognition systems have shown significantly higher error rates for people of color and women.
Lesson learned: Diverse representation in training data and development teams is crucial for creating fair and accurate AI systems.
Credit Scoring Discrimination
AI-powered credit scoring models have been found to disproportionately deny loans to minority applicants.
Lesson learned: Financial algorithms require rigorous testing and auditing to ensure fair treatment across all demographic groups.
The Future of AI: Balancing Innovation and Ethics
As AI continues to evolve and permeate various aspects of business and society, striking the right balance between innovation and ethical considerations will be crucial:
Ethical AI Development
- Incorporating ethical considerations from the outset of AI projects
- Developing AI systems that align with human values and societal goals
Transparency and Explainability
- Working towards AI systems that can provide clear explanations for their decisions
- Balancing the need for transparency with the complexity of advanced AI algorithms
Collaborative Governance
- Fostering collaboration between industry, academia, and regulatory bodies
- Developing flexible governance frameworks that can adapt to technological advancements
Conclusion: A Call to Action
The Progress Software study serves as a wake-up call for organizations utilizing AI technologies. The prevalence of data bias and the lack of preparedness to address it pose significant risks to businesses and society at large.
Addressing AI bias requires a multifaceted approach:
- Implementing robust data governance and quality control measures
- Investing in tools and technologies for bias detection and mitigation
- Fostering a culture of ethical AI development and usage
- Engaging with regulatory efforts to establish responsible AI practices
- Maintaining human oversight and leveraging diverse perspectives in AI development
By taking proactive steps to address data bias, organizations can harness the power of AI while mitigating its potential negative impacts. The future of AI lies not just in its technological capabilities, but in our ability to develop and deploy it responsibly, ensuring that it serves as a force for positive change in business and society.
As we move forward in this AI-driven era, it's imperative that businesses, technologists, and policymakers work together to create AI systems that are not only powerful and efficient but also fair, transparent, and aligned with human values. Only then can we truly realize the transformative potential of AI while safeguarding against its hidden risks.
Article created from: https://www.youtube.com/watch?v=-SNxyrca9Ac