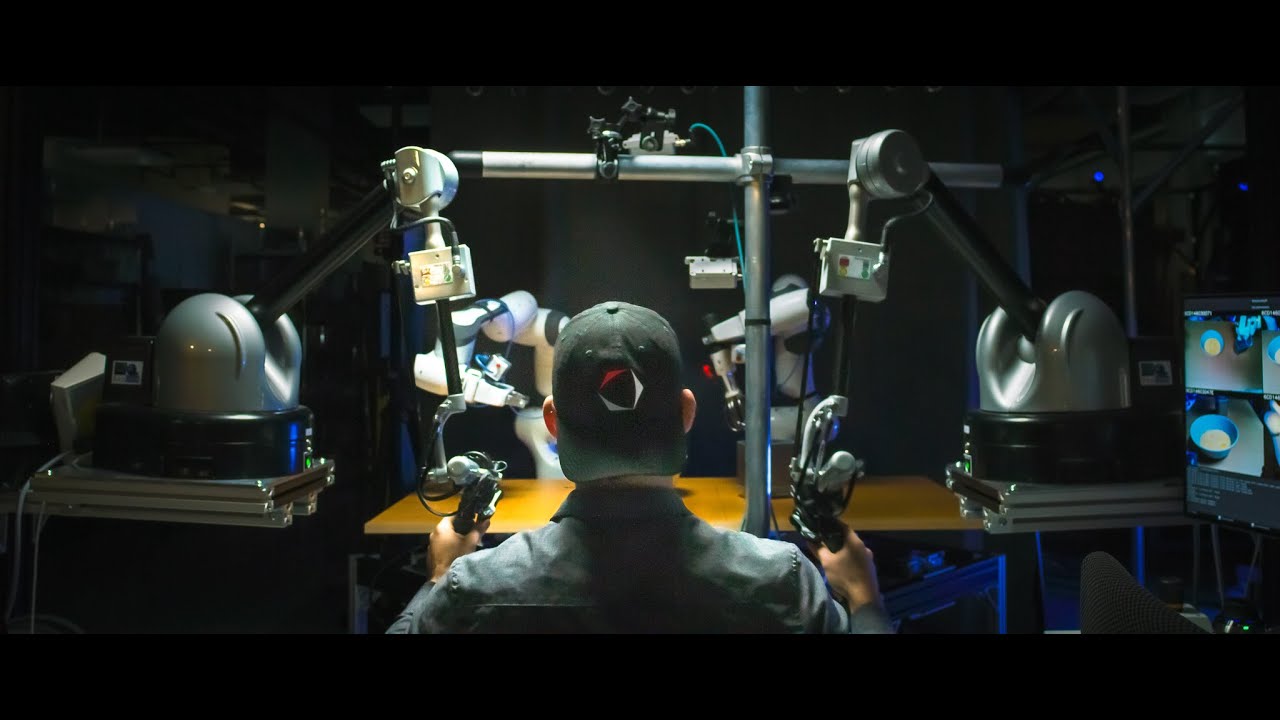
Create articles from any YouTube video or use our API to get YouTube transcriptions
Start for freeThe Dawn of Dexterous Robots: A Leap Forward with Generative AI
In the rapidly evolving field of robotics, a groundbreaking breakthrough promises to amplify human capabilities at home and in the workplace like never before. The key to this revolution? A novel method known as diffusion policy, a potent generative AI technique that is reshaping how robots learn and interact with their environments.
Unlocking New Possibilities
Until recently, robotic manipulation largely centered around simple pick-and-place tasks, limiting robots to a narrow range of actions. However, the advent of diffusion policy has dramatically expanded these horizons. Robots are now mastering complex tasks such as using tools, pouring liquids, and even peeling vegetables. This leap in capability is achieved without rewriting code or explicitly programming new skills, marking a significant advancement in robotic dexterity and autonomy.
The Magic of Diffusion Policy
At the heart of this transformation is the diffusion policy technique. By leveraging powerful generative AI, researchers can teach robots a wide array of dexterous skills quickly and with fewer demonstrations than ever before. This method not only accelerates the learning process but also paves the way for creating large behavior models. These models hold the promise of revolutionizing robotics in the same way that large language models have transformed chatbots.
From Teaching to Learning Overnight
The process begins with a human teacher demonstrating a skill through teleoperation. In a matter of hours, the AI-based diffusion policy processes these demonstrations, enabling robots to learn and perfect a new behavior overnight. This rapid learning cycle is a game-changer, allowing for the continuous expansion of a robot's skill set.
The Importance of Touch
A critical factor in this learning process is the sense of touch. Providing robots with haptic feedback, akin to human tactile sensation, significantly enhances their ability to learn. Tasks that require a delicate touch, such as flipping a pancake, become achievable as robots can feel their interaction with objects and surfaces, mirroring the human learning experience.
Looking Ahead: The Future of Robot Learning
The journey towards large behavior models is just beginning. The ultimate goal is to train robots with an extensive array of dexterous skills, enabling them to generalize and perform tasks they have never been explicitly taught. This vision involves creating a diverse curriculum for robot learning, effectively a 'kindergarten for robots,' where foundational skills vital for working alongside humans are imparted.
With plans to teach hundreds of new behaviors by the end of the year and over a thousand by the end of 2024, the future of robotics is incredibly promising. The use of simulation to augment real-world teaching and the development of tools for fleet learning—wherein when one robot learns a skill, all others learn it too—further accelerates this progress.
A Robotic Renaissance
The pace at which robots are acquiring new skills is unprecedented. For those involved in robotics research, witnessing these advancements firsthand is nothing short of astonishing. Nearly every day brings news of robots accomplishing tasks that were previously unimaginable, signaling a renaissance in the field of robotics.
This exciting era of innovation is not just transforming the capabilities of robots but is also redefining the potential for robotic assistance in our daily lives, both at home and in the workplace. As we stand on the brink of this new frontier, one thing is clear: the future of robotics, powered by generative AI and diffusion policy, is here.
For more insights into this transformative research in robotics, watch the full video here.