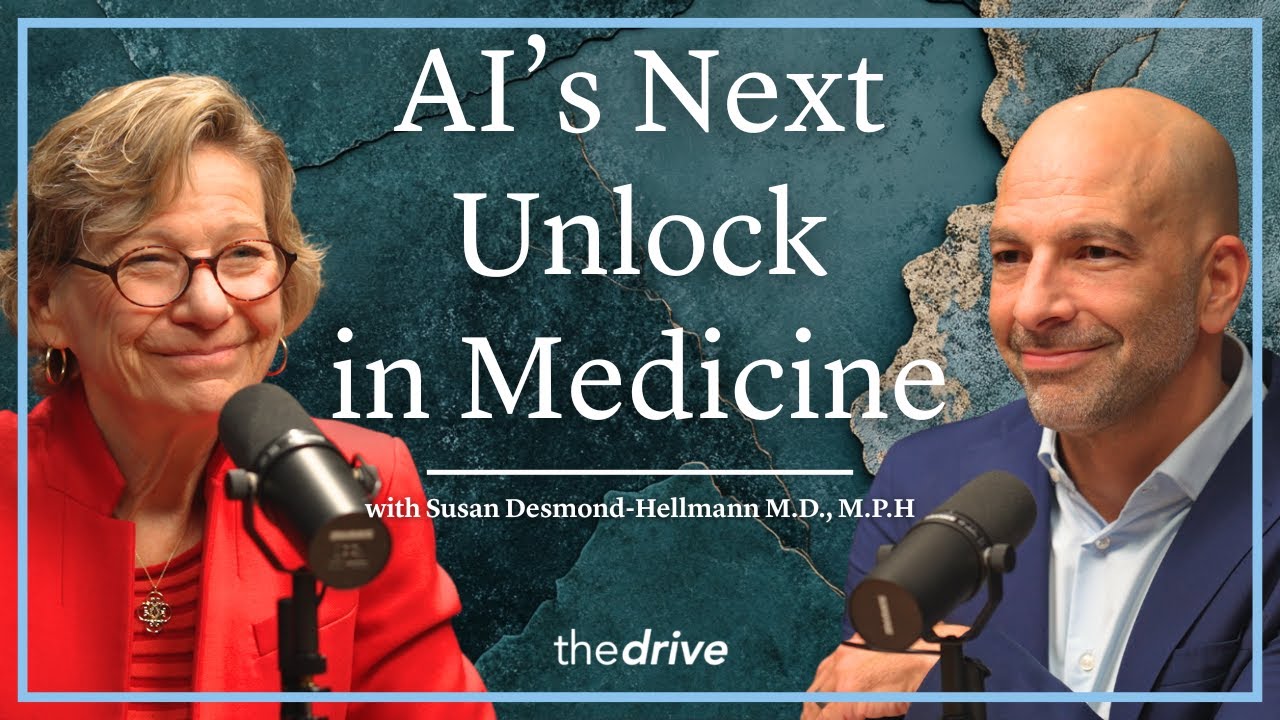
Create articles from any YouTube video or use our API to get YouTube transcriptions
Start for freeThe Nobel Prize-Winning Breakthrough in Protein Folding
In recent years, artificial intelligence (AI) has made significant strides in various fields, but perhaps none as impactful as its application in medicine and biotechnology. One of the most notable achievements in this area was recognized by the Nobel Prize committee, which awarded the prestigious honor for AI-driven protein folding analysis.
This groundbreaking work has opened up new possibilities in the field of biotechnology and has the potential to accelerate drug discovery and development processes dramatically. But what exactly does this mean for the future of medicine, and what challenges lie ahead?
Understanding the Significance of AI-Driven Protein Folding Analysis
Protein folding is a complex process that determines the three-dimensional structure of proteins, which in turn dictates their function in living organisms. Predicting how proteins fold has been a longstanding challenge in biology, with immense implications for understanding diseases and developing new treatments.
The AI-driven approach to protein folding analysis has revolutionized this field by:
- Accelerating the prediction process
- Improving accuracy
- Enabling the study of previously intractable protein structures
- Providing insights into protein function and interactions
This breakthrough is particularly significant in the pre-clinical stage of drug development. By rapidly and accurately predicting protein structures, researchers can:
- Identify potential drug targets more efficiently
- Design more effective and targeted therapies
- Reduce the time and cost associated with early-stage drug discovery
The Impact on Biotechnology and Medicine
The implications of this AI-driven breakthrough extend far beyond the laboratory. Here are some ways in which it is likely to advance biotechnology and medicine:
-
Faster Drug Development: By streamlining the initial stages of drug discovery, AI-powered protein folding analysis can significantly reduce the time it takes to bring new treatments to market.
-
Personalized Medicine: A better understanding of protein structures and interactions can lead to more tailored treatments based on an individual's genetic makeup.
-
Novel Therapeutic Approaches: Insights gained from protein folding analysis may reveal new therapeutic strategies for previously untreatable diseases.
-
Improved Vaccine Design: The technology could accelerate the development of vaccines by providing a clearer picture of viral protein structures.
-
Advancements in Structural Biology: The ability to predict protein structures more accurately will enhance our overall understanding of cellular processes and disease mechanisms.
The Next Frontier: AI in Clinical Trials and Biomarkers
While the protein folding breakthrough represents a significant milestone, there are still many challenges ahead in the field of medicine and biotechnology. One area where AI could potentially make a substantial impact is in clinical trials and the development of biomarkers.
Shortening Clinical Trials with AI
Clinical trials are a crucial but time-consuming and expensive part of drug development. AI has the potential to streamline this process in several ways:
-
Patient Selection: AI algorithms can help identify the most suitable candidates for clinical trials, potentially reducing the number of participants needed and increasing the likelihood of success.
-
Data Analysis: Machine learning techniques can process vast amounts of data from trials more quickly and accurately than traditional methods.
-
Predictive Modeling: AI can help predict potential outcomes and side effects, allowing researchers to make more informed decisions throughout the trial process.
-
Real-time Monitoring: AI-powered systems can continuously monitor trial data, flagging potential issues or promising results earlier in the process.
The Quest for Better Biomarkers
One of the most promising areas for AI application in medicine is the development and utilization of biomarkers. Biomarkers are measurable indicators of biological states or conditions, and they play a crucial role in diagnosis, treatment selection, and monitoring disease progression.
The ideal biomarker would be:
- Highly specific to a particular condition
- Easily measurable
- Responsive to changes in the disease state or treatment efficacy
AI could potentially help identify new biomarkers and improve the use of existing ones by:
- Analyzing large datasets to identify patterns and correlations
- Integrating multiple types of data (genetic, proteomic, metabolomic, etc.) to create more comprehensive biomarker profiles
- Developing more sensitive and specific measurement techniques
- Predicting the most useful biomarkers for specific conditions or patient populations
The Power of Viral Load Measurements
An excellent example of the impact of effective biomarkers is the use of viral load measurements in HIV treatment. This approach allowed for the rapid development of numerous HIV drugs in a relatively short period. The success of viral load as a biomarker stems from its ability to provide a clear, quantifiable measure of treatment efficacy.
Applying this concept to other diseases could potentially revolutionize treatment approaches. For instance, if we could develop similarly effective biomarkers for various types of cancer, it could lead to more targeted and effective treatments.
The Challenge of Early Cancer Detection
One area where improved biomarkers and AI analysis could make a significant impact is in early cancer detection. Currently, early detection methods are limited and not equally effective across all types of cancer.
Current Successful Early Detection Methods
Some of the most effective early detection methods currently available include:
- Colonoscopy for colorectal cancer
- Pap smears for cervical cancer
- HPV vaccination for preventing cervical cancer
- Spiral CT scans for lung cancer
- PSA testing for prostate cancer (when used appropriately)
However, these methods cover only a small fraction of cancer types, and many have limitations in terms of accuracy, invasiveness, or cost.
The Potential of Liquid Biopsies
Liquid biopsies, which involve analyzing blood samples for cancer biomarkers, have been proposed as a potential solution for early cancer detection across multiple cancer types. However, current liquid biopsy techniques face significant challenges, particularly in terms of sensitivity.
The main obstacle appears to be that many tumors don't shed enough DNA into the bloodstream to be reliably detected, especially in early stages. This limitation has led to skepticism about the current effectiveness of liquid biopsies for early cancer detection.
However, AI could potentially help address some of these challenges by:
- Improving the sensitivity of detection methods
- Integrating multiple types of biomarkers (DNA, RNA, proteins) for more accurate results
- Developing more sophisticated algorithms for interpreting liquid biopsy data
The Case of Breast Cancer
Breast cancer presents a particular challenge for early detection due to its complex progression pattern. Unlike colorectal cancer, which typically follows a clear progression from polyps to cancer, breast cancer development is less straightforward.
If a reliable early detection method for breast cancer could be developed, it would have a tremendous impact on treatment outcomes. Some experts suggest that protein-based biomarkers might hold promise for early breast cancer detection, but significant research is still needed in this area.
The Role of AI in Advancing Medicine
As we look to the future of medicine and biotechnology, it's clear that AI will play an increasingly important role. From protein folding analysis to clinical trial optimization and biomarker discovery, AI has the potential to accelerate research, improve patient outcomes, and transform our approach to healthcare.
However, it's important to remember that AI is a tool, not a magic solution. Its effectiveness will depend on the quality of data it's trained on, the expertise of the researchers and clinicians using it, and our ability to integrate AI-driven insights into clinical practice.
Some key areas where AI is likely to make significant contributions in the coming years include:
-
Drug Discovery and Development: AI will continue to accelerate the process of identifying potential drug targets and optimizing drug candidates.
-
Personalized Medicine: By analyzing vast amounts of patient data, AI can help tailor treatments to individual genetic profiles and disease characteristics.
-
Diagnostic Imaging: AI algorithms are already improving the accuracy and speed of interpreting medical images, and this trend is likely to continue.
-
Electronic Health Records: AI can help make sense of the enormous amount of data contained in electronic health records, identifying patterns and predicting health outcomes.
-
Robotic Surgery: AI-powered surgical robots could enhance precision and reduce recovery times for patients.
-
Mental Health: AI chatbots and analysis tools could help in early detection and management of mental health conditions.
-
Epidemic Prediction and Management: AI models can analyze global health data to predict and track the spread of infectious diseases.
Challenges and Ethical Considerations
While the potential of AI in medicine is enormous, it's crucial to address the challenges and ethical considerations that come with its implementation:
-
Data Privacy and Security: As AI relies on large datasets, ensuring the privacy and security of patient data is paramount.
-
Bias in AI Algorithms: AI systems can perpetuate or even amplify existing biases in healthcare if not carefully designed and monitored.
-
Interpretability: Many AI systems, especially deep learning models, operate as "black boxes," making it difficult to understand how they arrive at their conclusions. This lack of interpretability can be problematic in medical contexts.
-
Integration with Existing Systems: Implementing AI solutions in healthcare settings requires careful integration with existing systems and workflows.
-
Regulatory Challenges: The rapid pace of AI development often outstrips the ability of regulatory bodies to create appropriate guidelines and standards.
-
Ethical Decision-Making: As AI systems become more involved in medical decision-making, we must grapple with complex ethical questions about autonomy, responsibility, and the role of human judgment.
Conclusion
The Nobel Prize-winning breakthrough in AI-driven protein folding analysis marks a significant milestone in the application of artificial intelligence to medicine and biotechnology. This achievement, along with ongoing advancements in areas such as clinical trials, biomarker discovery, and early disease detection, points to a future where AI plays an increasingly central role in healthcare.
However, realizing the full potential of AI in medicine will require ongoing research, careful consideration of ethical implications, and a commitment to integrating these new technologies into existing healthcare systems in a way that truly benefits patients.
As we move forward, it's clear that the intersection of AI and medicine will continue to be a fertile ground for innovation, offering the promise of more effective treatments, earlier disease detection, and ultimately, better health outcomes for people around the world.
The journey from the laboratory to the clinic is often long and challenging, but with AI as a powerful ally, we may be able to navigate this path more quickly and effectively than ever before. The future of medicine is likely to be shaped by our ability to harness the power of AI while maintaining the human touch that is so essential to healthcare.
Article created from: https://youtu.be/_dc9d22Oeio?feature=shared