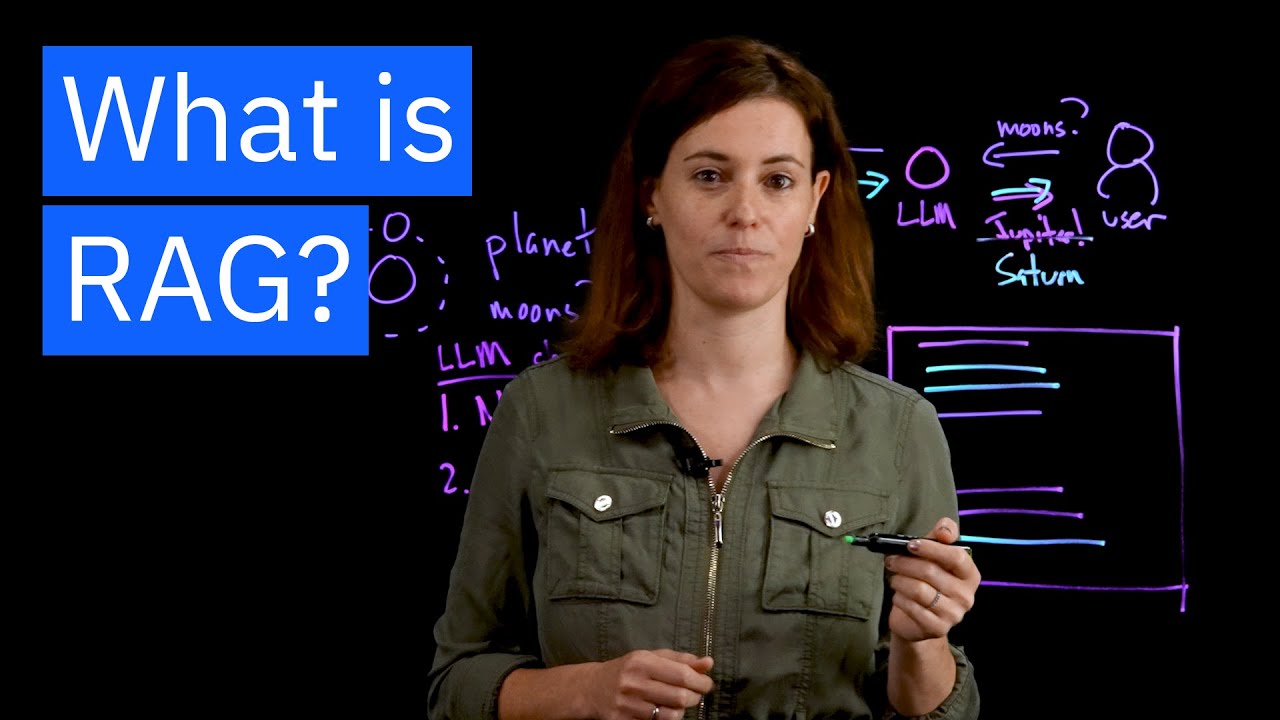
Create articles from any YouTube video or use our API to get YouTube transcriptions
Start for freeThe Evolution of Large Language Models with RAG
In the realm of artificial intelligence, large language models (LLMs) have become ubiquitous, providing answers to myriad user queries with varying degrees of accuracy. Despite their intelligence, these models are not without their shortcomings, often delivering responses that are either out-of-date or unsupported by credible sources. Marina Danilevsky, a Senior Research Scientist at IBM Research, introduces a promising solution to these issues: the Retrieval-Augmented Generation (RAG) framework. This innovative approach aims to significantly enhance the reliability and currency of information provided by LLMs.
Understanding the Generation in LLMs
Before diving into the intricacies of RAG, it's important to grasp what 'generation' entails in this context. Generation refers to the process wherein LLMs produce text in response to a prompt or query from users. While impressive, this capability isn't flawless. Anecdotal evidence, such as a personal story shared by Danilevsky about a query regarding the planet with the most moons in our solar system, highlights how reliance on outdated information can lead to incorrect answers.
The Pitfalls of Current LLMs
Danilevsky's anecdote underscores two primary challenges faced by LLMs:
- Lack of Credible Sources: Often, LLMs provide answers based solely on the data they were trained on, without citing current or credible sources.
- Outdated Information: The data LLMs were trained on can become outdated, leading to incorrect responses to queries about dynamic or evolving subjects.
Introducing Retrieval-Augmented Generation (RAG)
RAG addresses these issues head-on by incorporating a 'retrieval' phase before the 'generation' of a response. This means that an LLM, upon receiving a query, first consults a content store (which can be an open internet source or a closed document collection) to fetch the most relevant and up-to-date information. Only then does it generate a response, significantly improving the accuracy and relevancy of the answer.
The Three-Step Process of RAG
The RAG framework modifies the traditional response generation process as follows:
- User Query: The user presents a question to the LLM.
- Retrieval of Relevant Content: Instead of generating an answer immediately, the LLM retrieves relevant information from a content store.
- Informed Response Generation: The LLM combines the retrieved information with the original query to produce a more accurate and evidence-based answer.
Addressing the Challenges with RAG
RAG directly combats the two main challenges identified earlier:
- Keeping Information Current: By continuously updating the content store with new data, RAG ensures that LLMs have access to the most current information, eliminating the problem of outdated answers.
- Credibility and Evidence: By retrieving information from reputable sources before generating a response, RAG enables LLMs to provide answers that are not only accurate but also verifiable, reducing the likelihood of 'hallucinating' answers or leaking sensitive information.
The Future of RAG in AI
Despite its advantages, the efficacy of RAG is contingent on the quality of both the retriever and the generated response. This is why researchers, including those at IBM, are tirelessly working to enhance both components of the RAG framework. The goal is to ensure that LLMs can deliver the most accurate, reliable, and nuanced answers possible, ultimately fostering a more trustworthy interaction between AI and users.
As we continue to delve into the possibilities offered by RAG and other advancements in AI, it's clear that the journey towards perfecting large language models is far from over. However, with each innovation, we come one step closer to creating AI systems that can truly understand and respond to our needs with the precision and accuracy we desire.
To learn more about the revolutionary impact of Retrieval-Augmented Generation on large language models, watch Marina Danilevsky's insightful presentation here: IBM Research on RAG.