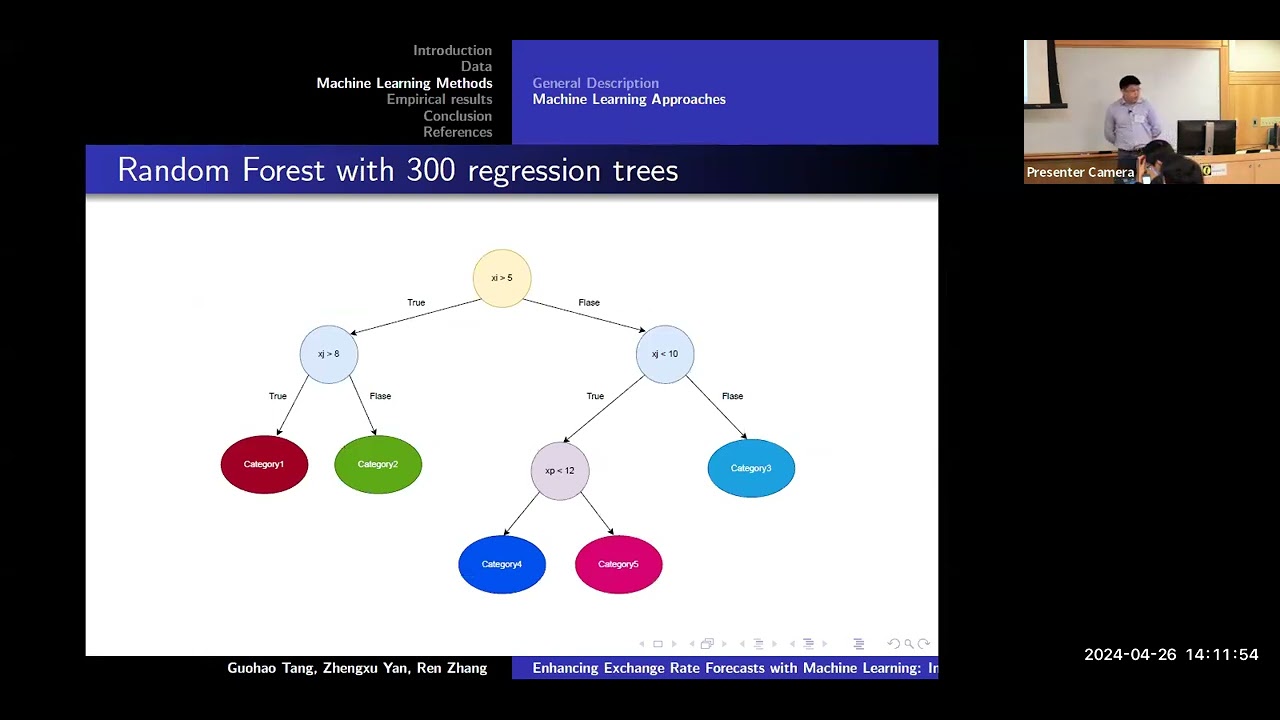
Create articles from any YouTube video or use our API to get YouTube transcriptions
Start for freeIntroduction
Forecasting exchange rates has long been a challenging task in international finance. Traditional models using macroeconomic variables have struggled to outperform simple random walk forecasts, leading to the well-known "exchange rate disconnect puzzle". This study explores a novel approach to exchange rate prediction by leveraging machine learning techniques and firm-level financial characteristics.
Motivation and Approach
The researchers hypothesize that firm-level data may incorporate relevant information for exchange rate movements more effectively than official macroeconomic releases. Their approach aims to address two key limitations of traditional models:
- Overly simplistic models with a limited number of variables
- Low-frequency macroeconomic data subject to reporting delays and revisions
By utilizing machine learning methods and a large set of firm characteristics, the study seeks to capture complex non-linear relationships and leverage higher-frequency financial data.
Methodology
The research forecasts exchange rates for 13 currencies over short-term horizons of 1 month and 6 months. It employs 153 firm financial characteristics as predictive variables, testing three machine learning approaches:
- Elastic Net
- Random Forest
- Support Vector Machines
The study aims to answer several key questions:
- Do machine learning approaches with firm characteristics forecast better than random walk benchmarks?
- Which machine learning method performs best?
- What types of firm characteristics have predictive power for exchange rates?
- Can the forecasts generate economic value through portfolio construction?
- Which components of exchange rate movements (interest differentials, risk premia, or liquidity premia) are most predictable using firm characteristics?
Data and Variables
The dataset covers the period from January 2005 to December 2020. The dependent variable is the log growth rate of exchange rates. Independent variables consist of 153 firm-level financial characteristics derived from Compustat data, reorganized based on a 2023 Journal of Finance paper.
The study uses approximately 20,000 firms, though the distribution varies across countries. Forecasts are conducted on a country-by-country basis, using only firms from the relevant country for each currency prediction.
Machine Learning Methods
Elastic Net
A combination of L1 (Lasso) and L2 (Ridge) regularization, allowing for fine-tuning of parameters to achieve optimal forecasts.
Random Forest
Utilizes 300 regression trees for each country, with trees up to 13 layers deep to generate predictions.
Support Vector Machines
Maps observations to higher-dimensional space to improve separability and predictive power.
Key Findings
Predictive Accuracy
The study evaluates predictive accuracy using out-of-sample R-squared and Clark and West mean squared forecast error tests. Key results include:
- For 1-month forecast horizons, machine learning methods outperform random walk for most currencies, with exceptions being the Australian dollar, Canadian dollar, and Swedish krona.
- At 6-month horizons, machine learning approaches beat random walk for all currencies studied.
- Random Forest generally performs best, though Support Vector Machines excel for some currencies (Swiss franc, South Korean won, Russian ruble).
Important Firm Characteristics
The research categorizes firm characteristics into six groups:
- Momentum
- Value
- Investment
- Profitability
- Intangibles
- Trading frictions
Findings on characteristic importance include:
- Investment characteristics are dominant predictors for the US dollar and South African rand.
- Investment features are important for 10 out of 13 currencies.
- Value characteristics are only important for 3 currencies.
- Significant heterogeneity exists in important characteristics across currencies.
Economic Value of Forecasts
The study constructs currency portfolios based on the forecasts to assess economic value:
- Machine learning-based portfolios achieve Sharpe ratios of 1.8-1.9, compared to 1.4 for random walk forecasts.
- Cumulative returns from 2007 onward show significantly higher gains using machine learning methods versus random walk assumptions.
- The three machine learning approaches yield similar economic performance.
Implications and Future Work
This research demonstrates the potential for machine learning techniques and firm-level financial data to improve exchange rate forecasting. The findings challenge the exchange rate disconnect puzzle and offer new avenues for currency prediction and investment strategies.
Future work will focus on several areas:
- Exploring links between firm characteristics and theoretical exchange rate determinants (interest rate differentials, risk premia, liquidity premia).
- Testing robustness using alternative benchmark currencies (e.g., US dollar instead of euro).
- Investigating whether improved exchange rate forecasts can help explain other macroeconomic variables.
Addressing Potential Concerns
Timeliness of Firm-Level Data
One might question the use of firm characteristics given potential reporting lags. The researchers offer several justifications:
- Diverse reporting schedules across thousands of firms provide frequent information updates.
- Firm data is less subject to revisions compared to some macroeconomic releases.
- Empirical results validate the additional informational content despite potential lags.
Short-Term vs. Long-Term Forecasting
The study focuses on what finance considers short-term horizons (1 and 6 months). Results suggest machine learning approaches are effective at both horizons, with some indication that 6-month forecasts may be stronger. However, both timeframes are considered short-term from a macroeconomic perspective.
Conclusion
This innovative approach to exchange rate forecasting demonstrates the potential for machine learning techniques and firm-level financial data to outperform traditional models. By leveraging a rich set of firm characteristics and advanced predictive methods, the study offers new insights into currency movements and challenges long-standing puzzles in international finance.
The research opens up exciting possibilities for both academic exploration and practical applications in currency trading and risk management. As the field continues to evolve, integrating machine learning with financial theory and firm-level data may lead to further breakthroughs in our understanding and prediction of exchange rate dynamics.
Article created from: https://www.youtube.com/watch?v=ryt-ccm96HM