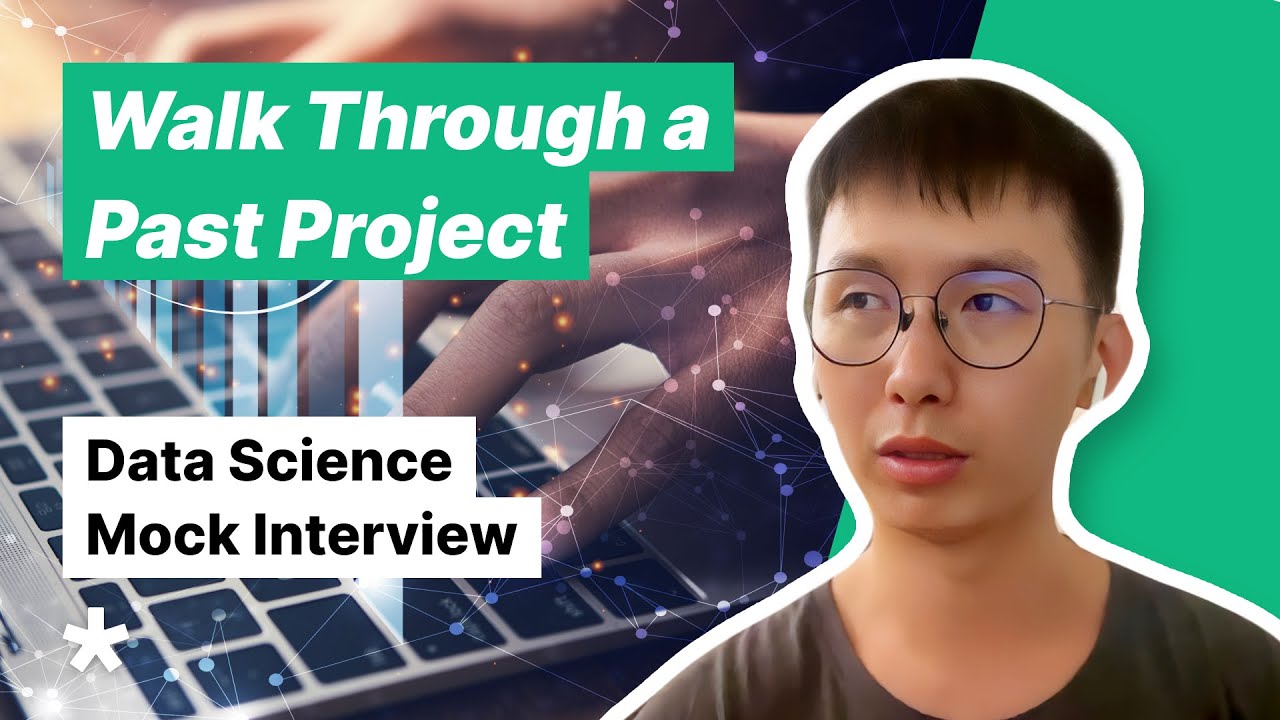
Create articles from any YouTube video or use our API to get YouTube transcriptions
Start for freeIntroduction
Email marketing remains one of the most cost-effective channels for e-commerce businesses to engage customers and drive sales. However, many companies struggle to fully leverage the power of data and analytics to optimize their email campaigns. In this article, we'll explore how one data scientist revolutionized their company's approach to email marketing, resulting in a significant boost to conversion rates and overall sales contribution.
Setting the Foundation: Building Analytical Support
When tasked with improving the company's email marketing efforts, the data scientist's first step was to build analytical support from the ground up. This involved several key steps:
Stakeholder Collaboration
The data scientist began by meeting with product managers and the marketing team to:
- Discuss expectations for the email marketing channel
- Define the channel's strategic position within the overall marketing mix
- Assess available data sources and quality
- Finalize key metrics for reporting and analysis
Data Pipeline Development
Working closely with data engineers, the next phase focused on:
- Evaluating data quality across relevant sources
- Building robust data pipelines to ensure reliable, timely data flow
- Implementing data quality checks and monitoring
Dashboard Creation
With a solid data foundation in place, the data scientist proceeded to build a comprehensive dashboard using Tableau and SQL. Key features of the dashboard included:
- Weekly and monthly views of performance metrics
- Customizable filters for detailed analysis
- Self-service reporting capabilities for business teams
Key Metrics for Email Marketing Success
The data scientist implemented a funnel-based approach to measure email marketing performance, focusing on the following core metrics:
Open Rate
- Calculated as: (Number of emails opened) / (Number of emails sent)
- Measures the effectiveness of subject lines and sender reputation
Click-Through Rate (CTR)
- Calculated as: (Number of clicks) / (Number of emails opened)
- Indicates the relevance and appeal of email content and calls-to-action
Conversion Rate
- Calculated as: (Number of purchases) / (Number of email clicks)
- Measures the ultimate effectiveness of email campaigns in driving sales
Behavioral Analysis and Optimization
With the dashboard providing clear visibility into performance metrics, the data scientist conducted in-depth behavioral analysis to uncover optimization opportunities. One key finding emerged from analyzing shopping cart reminder emails:
Shopping Cart Reminder Email Insight
The data scientist discovered that customers who made purchases after receiving a cart reminder email often bought products in the same category as those left in their cart, rather than the exact items they had abandoned.
Recommendation Engine Implementation
Based on this insight, the data scientist proposed adding a product recommendation section to cart reminder emails. This new element would showcase similar items to those in the customer's cart, potentially increasing the likelihood of a purchase.
Implementing A/B Testing
To validate the effectiveness of the new recommendation feature, the data scientist set up a rigorous A/B testing framework:
Hypothesis
Adding a product recommendation list to cart reminder emails will increase the conversion rate from shopping cart to checkout by at least 5%.
Experimental Setup
- Control Group: Received the standard cart reminder email
- Treatment Group: Received the modified email with product recommendations
- Sample Size: Calculated based on desired statistical power (80%) and significance level (α = 0.05)
- Test Duration: 3 weeks (based on weekly email volume and required sample size)
Statistical Analysis
- Primary Metric: Conversion rate (shopping cart to checkout)
- Statistical Test: Independent samples t-test
- Assumption: Conversion rate follows a normal distribution
Guardrail Metrics
To ensure the new email design didn't negatively impact other important factors, the following guardrail metrics were monitored:
- Average items added to cart per user
- Email click-through rate
Results and Impact
The A/B test yielded positive results, confirming the effectiveness of the new recommendation feature:
- The treatment group showed a statistically significant increase in conversion rate
- Guardrail metrics remained stable or showed slight improvements
- Overall email marketing contribution to sales increased by 30% quarter-over-quarter for two consecutive quarters
- Email marketing's share of total sales grew from 3% to 7% within six months
Expanding the Data-Driven Approach
Building on the success of the initial A/B test, the data scientist implemented a more sophisticated testing and optimization framework:
Segmentation and Attribution Analysis
Further tests incorporated user segmentation to uncover insights specific to different user groups:
- New users vs. returning customers
- iOS vs. Android device users
- Different email client interfaces
This granular analysis revealed that some changes had neutral overall effects but significantly improved metrics for specific user segments.
Advanced Traffic Allocation
To maximize the impact of successful variations, the team moved beyond the traditional 50/50 split for A/B tests:
- Implemented 25/75 traffic splits for promising new variations
- Adopted Bayesian statistical methods to support non-equal traffic allocation
- Allowed for faster iteration and learning while maintaining statistical rigor
Bayesian vs. Frequentist Approach in A/B Testing
The shift from a frequentist to a Bayesian approach in A/B testing brought several advantages:
Advantages of Bayesian A/B Testing
-
Flexibility in sample sizes: Bayesian methods don't require a predetermined sample size, allowing for more dynamic decision-making.
-
Intuitive interpretation: Results are expressed as probabilities, making them easier for non-technical stakeholders to understand.
-
Incorporation of prior knowledge: Bayesian methods allow the inclusion of existing knowledge or beliefs about the test variables.
-
Continuous monitoring: Decisions can be made as soon as sufficient evidence is gathered, without waiting for a fixed test duration.
-
Better handling of small sample sizes: Bayesian methods can provide more reliable insights when working with limited data.
Implementation of Bayesian Testing
The data scientist implemented Bayesian A/B testing using the following approach:
-
Define priors: Establish initial beliefs about the conversion rates for each variation based on historical data and expert knowledge.
-
Collect data: Run the test, continuously updating the posterior distributions as new data comes in.
-
Calculate probabilities: Determine the probability that each variation is the best performer.
-
Make decisions: Use predefined decision thresholds (e.g., 95% probability of being the best) to conclude tests and implement winners.
Scaling Success: Applying Learnings Across the Organization
The success of the data-driven approach in email marketing led to broader adoption across the company:
Knowledge Sharing
- The data scientist presented findings and methodologies to the larger data science and product teams.
- Best practices for A/B testing and Bayesian analysis were documented and shared.
Cross-Functional Collaboration
- The email marketing team partnered with other departments to identify additional optimization opportunities.
- The data-driven culture spread, encouraging more teams to adopt rigorous testing practices.
Continuous Improvement
- The team committed to running at least 10 A/B tests per quarter to drive ongoing optimization.
- Regular reviews of test results and insights informed future hypotheses and experiments.
Key Takeaways for Data Scientists
This case study offers several valuable lessons for data scientists working on similar projects:
-
Start with a solid foundation: Invest time in building robust data pipelines and dashboards to enable data-driven decision-making.
-
Collaborate closely with stakeholders: Regular communication with product and marketing teams ensures alignment on goals and metrics.
-
Think beyond technical solutions: Combine data analysis with business acumen and competitive research to drive meaningful insights.
-
Embrace iterative testing: Use A/B testing to validate hypotheses and continuously improve performance.
-
Consider advanced statistical methods: Explore techniques like Bayesian analysis to gain deeper insights and make more informed decisions.
-
Focus on actionable insights: Translate data findings into clear, actionable recommendations for business teams.
-
Scale successes: Share learnings and best practices across the organization to maximize impact.
Conclusion
By implementing a data-driven approach to email marketing optimization, this data scientist not only significantly improved the performance of a key marketing channel but also fostered a culture of experimentation and continuous improvement within the organization. The combination of rigorous analysis, strategic A/B testing, and advanced statistical methods proved to be a powerful formula for driving meaningful business results.
As e-commerce continues to evolve, the ability to leverage data effectively will become increasingly crucial for companies looking to stay competitive. By following the principles and methodologies outlined in this case study, data scientists can play a pivotal role in driving growth and innovation across various business functions.
Remember, the journey to optimization is ongoing. Continue to question assumptions, test new ideas, and refine your approach based on data-driven insights. With persistence and creativity, you can unlock significant value for your organization through the power of data science.
Article created from: https://www.youtube.com/watch?v=c1dp2UhjO54&list=PLrtCHHeadkHqFEO69UiOj9BHA7pah-0fj&index=9