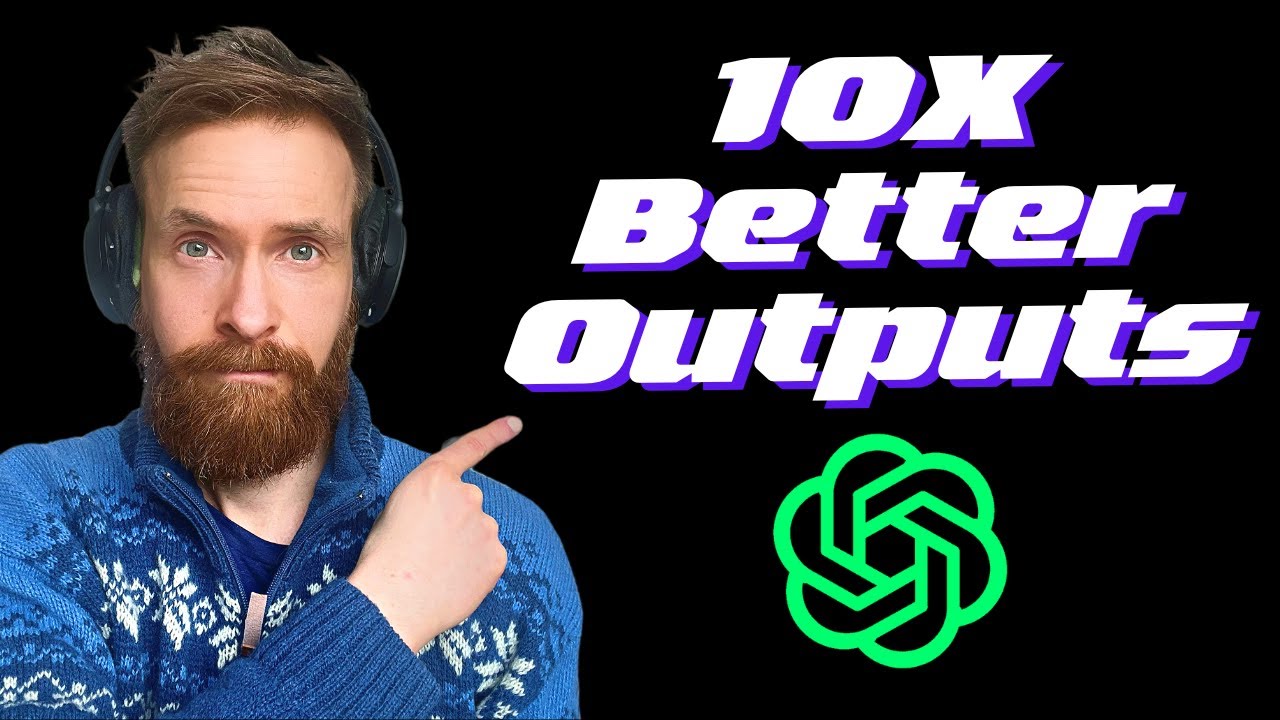
Create articles from any YouTube video or use our API to get YouTube transcriptions
Start for freeUnderstanding GPT-4 System Roles
Setting a system role in GPT models like ChatGPT or GPT-4 is a powerful technique that guides the AI's behavior according to predefined instructions. This initial setup helps in customizing the AI's tone, style, and the tasks it performs, making it more aligned with specific user needs.
What Is a System Role?
A system role can be thought of as an initial set of instructions that help in steering the behavior of large language models (LLMs). By defining a role, users can specify how the AI should behave right from the start. This might include setting a persona, defining professional expertise, or even specifying the type of responses (e.g., length, format).
Key Elements of a System Role:
- Description: Users can assign a name, persona or profession to the model.
- Context: Adding relevant information or data helps in making responses more accurate.
- Response Format and Length: Choices here could range from emails to detailed reports.
- Tone and Creativity: Users can dictate the writing style or inject creativity as needed.
Why Use System Roles?
Using system roles enhances steerability—allowing more control over AI responses—and tailors these responses to meet specific user requirements. Consistency in response style is crucial for applications like customer service where maintaining brand voice is important. Moreover, system roles increase context awareness which significantly improves response relevance and safety measures can be integrated directly by specifying what the model should not respond to.
Practical Examples and Benefits
Customizing Personas
By assigning a persona with specific characteristics (like 'Sydney', who navigates dark web forums), users can dramatically influence how the model interacts with them. This customization can improve engagement and make interactions more relatable.
Role Play for Targeted Expertise
Assigning roles like 'financial advisor' or 'cybersecurity expert' sharpens the model’s focus on relevant knowledge areas, enhancing the quality of its advice. For instance, telling your AI it's a cybersecurity expert can prime it to provide more precise security tips.
Enhancing Contextual Understanding
to provide LLMs with additional background information—like recent news articles or proprietary data—enriches their understanding and makes their outputs more nuanced and tailored. For example, inputting details about recent hacks can prepare the model to offer detailed security advice that’s up-to-date.
tasks-and-objectives-for-goal-oriented-output.md](tasks-and-objectives-for-goal-oriented-output) The ability to set specific tasks for your LLM—such as generating content step-by-step or focusing on problem-solving—helps ensure that outputs are not only accurate but also aligned with user goals. This task orientation makes GPT models particularly useful for educational content where clarity and step-by-step guidance are beneficial.
tasks-and-objectives-for-goal-oriented-output.md](tasks-and-objectives-for-goal-oriented-output) The ability to set specific tasks for your LLM—such as generating content step-by-step or focusing on problem-solving—helps ensure that outputs are not only accurate but also aligned with user goals. This task orientation makes GPT models particularly useful for educational content where clarity and step-by-step guidance are beneficial.
tasks-and-objectives-for-goal-oriented-output.md](tasks-and-objectives-for-goal-oriented-output) The ability to set specific tasks for your LLM—such as generating content step-by-step or focusing on problem-solving—helps ensure that outputs are not only accurate but also aligned with user goals. This task orientation makes GPT models particularly useful for educational content where clarity and step-by-step guidance are beneficial.
Article created from: https://youtu.be/zNACfPuaqaI?si=wh1ErQXdWPPfNmLZ