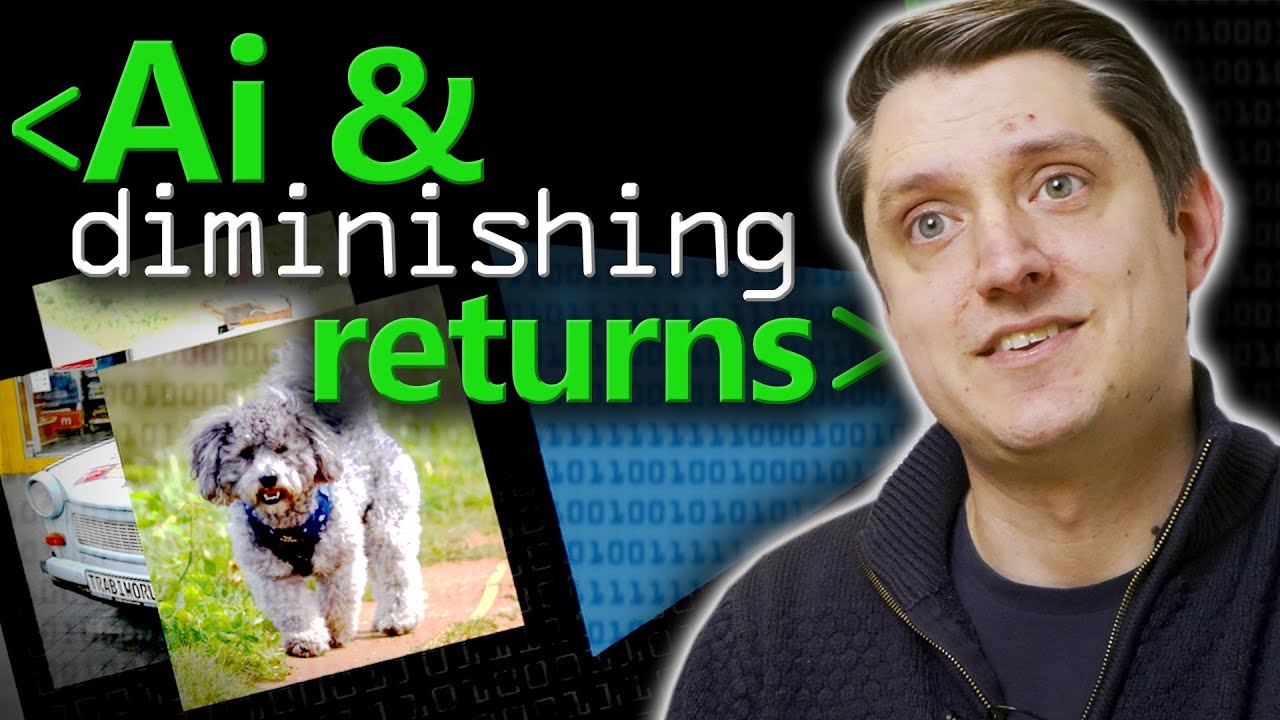
Create articles from any YouTube video or use our API to get YouTube transcriptions
Start for freeUnderstanding the Boundaries of AI Through Data Expansion
The rapid evolution of artificial intelligence has often been linked to the continuous enlargement of data sets and the development of more complex models. The prevailing belief in tech circles, particularly among major companies, is that by consistently feeding more data and creating larger models, AI can eventually achieve unparalleled general intelligence, capable of performing any task across various domains.
The Role of Clip Embeddings in AI Development
Clip embeddings have become a cornerstone in understanding how images can be translated effectively to textual descriptions through AI. By analyzing numerous image-text pairs, these systems learn to distill complex visual information and represent it linguistically. This process involves using large vision transformers paired with extensive text encoders to create a shared embedded space—a numerical fingerprint that captures the essence of both textual and visual inputs.
Challenging the Infinite Data Model Theory
Recent studies, however, challenge this optimistic view. A pivotal paper discussed in detail reveals that achieving general zero-shot performance—where an AI can handle tasks it has never seen before—requires an impractically vast amount of data. This finding suggests a potential plateau in performance improvements despite increasing data input, contradicting the notion that just adding more data or bigger models could solve all computational problems.
Practical Applications and Limitations
AI systems like those used in streaming services for recommendations demonstrate practical uses of clip embeddings. These systems analyze watched content to recommend new programs by finding similarities in their embedded spaces. However, when it comes to more complex tasks such as specific medical diagnoses or recognizing rare biological species, these models falter due to insufficient specific data.
Downstream Tasks and Their Feasibility
The effectiveness of downstream tasks like classification or recommendation systems heavily depends on the available data for each concept. The discussed paper illustrates this through graphs showing how performance varies with the number of examples per concept. While some common items like cats might be overrepresented in datasets, rarer entities like specific tree species are not—leading to poorer model performance on these items.
Future Directions for AI Research
The conclusion drawn from recent research points towards the necessity for innovative approaches beyond mere data accumulation. New strategies might involve rethinking machine learning algorithms or finding novel ways to represent data that do not solely rely on volume.
Implications for Ongoing AI Development
As we continue to push the boundaries of what AI can achieve, it becomes crucial to manage expectations about its capabilities realistically. While larger datasets and more sophisticated models offer certain improvements, they also require immense resources without guaranteeing proportional gains in performance across all tasks.
In summary, while generative AI continues to impress with its ability to handle straightforward tasks effectively, its application in more specialized or rare scenarios remains limited without significant breakthroughs in how we approach machine learning training and model development.
Article created from: https://www.youtube.com/watch?v=dDUC-LqVrPU&t=439s