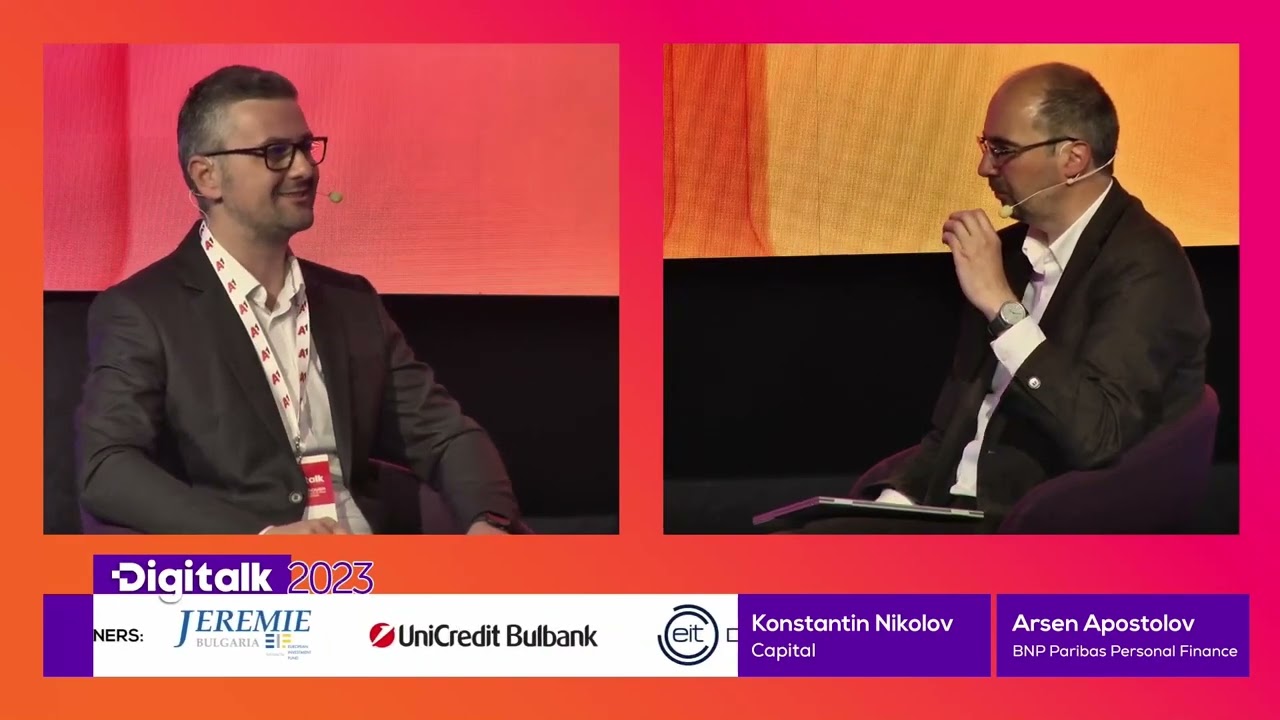
Create articles from any YouTube video or use our API to get YouTube transcriptions
Start for freeThe Reality of AI in Business: More Than Just Technology
In recent years, artificial intelligence (AI) has become a buzzword across industries, with many companies eager to implement AI solutions to stay competitive. However, the reality of AI implementation is far more nuanced than simply deploying cutting-edge technology. According to a leading expert in the field, the success of AI projects hinges on human input, careful planning, and a deep understanding of business needs.
The 80/20 Rule of AI Implementation
One of the most surprising insights shared by our expert is the distribution of effort in AI projects:
- 20% or less of the work involves technical implementation
- 80% of the effort is dedicated to communication and collaboration with stakeholders
This breakdown challenges the common perception that AI projects are primarily about coding and data science. In reality, the majority of time and resources are spent on:
- Organizing meetings with various departments
- Understanding specific use cases
- Helping business people envision potential solutions
- Designing implementation strategies
This human-centric approach is crucial for several reasons:
- It ensures that AI solutions address real business needs
- It helps manage expectations and avoid the "silver bullet" mentality
- It facilitates buy-in from all levels of the organization
- It leads to more practical and implementable solutions
The Importance of Clear Communication
Our expert emphasizes that even the most advanced AI models are useless if they cannot be explained to managers and end-users. The ability to communicate complex technical concepts in simple terms is a critical skill for anyone working on AI projects. This includes:
- Explaining how models work
- Clarifying the data inputs and outputs
- Demonstrating the business value of AI solutions
Without effective communication, AI projects risk remaining confined to development environments, never making it to production or delivering real value to the organization.
Real-World AI Use Cases in Personal Finance
To illustrate the practical applications of AI in the financial sector, our expert shared two compelling use cases from their organization.
Use Case 1: Price Optimization for Consumer Lending
One of the most impactful AI projects implemented by the company focused on price optimization for consumer lending products, including loans and credit cards. This use case demonstrates how AI can be applied to a critical business function that might not immediately come to mind when thinking about AI applications.
Key aspects of this project included:
- Collaboration across multiple departments (sales, marketing, risk management)
- Focus on a specific segment of the company's portfolio
- Careful design and implementation process
The results were impressive:
- Nearly 20% increase in financial impact for the targeted segment
- Achieved without simply raising prices
This case study highlights how AI can be used to optimize pricing strategies in ways that benefit both the company and its customers.
Use Case 2: Reducing Customer Service Costs
Another successful AI implementation addressed the challenge of high call volumes in customer service. While customer engagement is generally positive, excessive calls can lead to increased costs without necessarily improving customer satisfaction.
The AI project aimed to:
- Reduce costs associated with unnecessary calls
- Improve overall customer experience
The implementation process involved:
- Using an ensemble of AI techniques to identify the root causes of high call volumes
- Building predictive models to anticipate customer needs
- Analyzing the data to uncover unexpected insights
Key findings from the project:
- 25% of callers were generating 50% of the costs
- The AI model identified data points that initially seemed illogical to business users
- Further analysis of these data points revealed two internal processes that could be optimized
This use case demonstrates how AI can not only address the immediate problem (high call volumes) but also uncover deeper operational inefficiencies that might otherwise go unnoticed.
Best Practices for Implementing AI in Organizations
For companies looking to start their AI journey or improve their existing AI initiatives, our expert offers several key pieces of advice:
1. Start Small and Build Confidence
For organizations new to AI:
- Don't be intimidated by the complexity of AI
- Begin with a small, manageable project
- Focus on going through the full cycle of development, implementation, and evaluation
- Use initial successes to build confidence and support for larger initiatives
2. Collect and Centralize Data
Data is the foundation of any AI project. Organizations should:
- Map all data sources, including those outside traditional databases
- Consolidate data from various systems and partners
- Create a single source of truth for customer and account-level data
- Ensure data quality to avoid the "garbage in, garbage out" problem
3. Involve Stakeholders from Across the Organization
Successful AI implementation requires input from various departments:
- Gather representatives from all relevant areas of the business
- Brainstorm potential use cases for AI and analytics
- Prioritize projects based on potential impact and feasibility
- Use this process to develop a clear roadmap and budget for AI initiatives
4. Foster a Data Culture
Building a data-driven organization is a long-term endeavor:
- Implement ongoing initiatives to increase data literacy across all levels of the organization
- Educate employees on the possibilities and limitations of AI tools
- Ensure transparency in how data is used to improve operations and customer service
- Create internal tools (like the "instant data advisor" mentioned by our expert) to empower employees with data-driven insights
5. Embrace Continuous Learning and Improvement
AI implementation is not a one-time project but an ongoing process:
- Treat AI initiatives as a marathon, not a sprint
- Establish communities of practice to share knowledge and best practices
- Continuously evaluate and refine AI models and processes
- Stay informed about new developments in AI technology and applications
The Future of AI in Personal Finance
Looking ahead, our expert sees significant potential for AI to enhance customer experiences in personal finance. Some areas of focus include:
- Personal Finance Management tools powered by AI
- AI-driven budgeting and savings recommendations
- Intelligent product recommendations based on individual financial situations
These applications have the potential to not only improve operational efficiency for financial institutions but also to provide tangible benefits to customers in managing their finances more effectively.
Overcoming Challenges in AI Implementation
While the potential benefits of AI are significant, organizations must be prepared to address several challenges:
Data Privacy and Security
As AI systems rely heavily on personal and financial data, ensuring the privacy and security of this information is paramount. Organizations must:
- Implement robust data protection measures
- Comply with relevant regulations (e.g., GDPR, CCPA)
- Be transparent with customers about data usage
- Obtain necessary consents for data processing
Ethical Considerations
The use of AI in financial decision-making raises important ethical questions:
- How to ensure fairness and avoid bias in AI models
- Balancing automation with human judgment
- Maintaining accountability for AI-driven decisions
- Considering the societal impact of AI in finance
Technical Complexity
Despite advances in AI technology, implementing and maintaining AI systems remains complex:
- Acquiring and retaining skilled AI talent
- Keeping up with rapidly evolving AI technologies
- Integrating AI systems with existing IT infrastructure
- Scaling AI solutions across the organization
Change Management
Introducing AI can be disruptive to established processes and roles:
- Managing employee concerns about job displacement
- Retraining staff to work alongside AI systems
- Adapting organizational structures to support AI initiatives
- Overcoming resistance to change
Measuring the Success of AI Initiatives
To justify ongoing investment in AI, organizations need clear metrics for success. Some key performance indicators (KPIs) to consider include:
Financial Metrics
- Revenue growth attributable to AI initiatives
- Cost savings from AI-driven process improvements
- Return on investment (ROI) for AI projects
Operational Metrics
- Reduction in processing times
- Improvement in accuracy rates
- Increase in customer self-service adoption
Customer Experience Metrics
- Customer satisfaction scores
- Net Promoter Score (NPS)
- Reduction in customer churn
Employee Metrics
- Employee productivity improvements
- Job satisfaction among employees working with AI tools
- Adoption rates of AI-powered tools by staff
Building an AI-Ready Workforce
As AI becomes increasingly integral to business operations, organizations must focus on developing an AI-ready workforce. This involves:
Upskilling Existing Employees
- Providing training programs on AI concepts and applications
- Encouraging cross-functional learning and collaboration
- Offering opportunities for hands-on experience with AI tools
Recruiting AI Talent
- Developing partnerships with universities and research institutions
- Creating attractive career paths for AI specialists
- Fostering a culture of innovation to attract top talent
Promoting AI Literacy at All Levels
- Educating executives on AI strategy and governance
- Ensuring middle managers understand how to leverage AI in their departments
- Empowering front-line staff to work effectively with AI-powered tools
Conclusion: The Human Element in AI Success
As we've explored throughout this article, the successful implementation of AI in personal finance—and indeed in any industry—is not solely about technology. It's about people. From the initial stages of project planning to the ongoing process of refinement and improvement, human input and collaboration are essential.
Key takeaways include:
- AI projects require extensive communication and collaboration across departments.
- Starting small and building on successes is an effective strategy for AI adoption.
- Data management and centralization are crucial foundations for AI initiatives.
- Fostering a data-driven culture is a long-term commitment that pays dividends.
- The future of AI in personal finance lies in enhancing customer experiences and decision-making.
By focusing on these human-centric aspects of AI implementation, organizations can maximize the benefits of this powerful technology while avoiding common pitfalls. As AI continues to evolve, those companies that can effectively blend technological innovation with human insight and creativity will be best positioned to thrive in the rapidly changing landscape of personal finance and beyond.
Article created from: https://www.youtube.com/watch?v=eeO4gxu_7nc