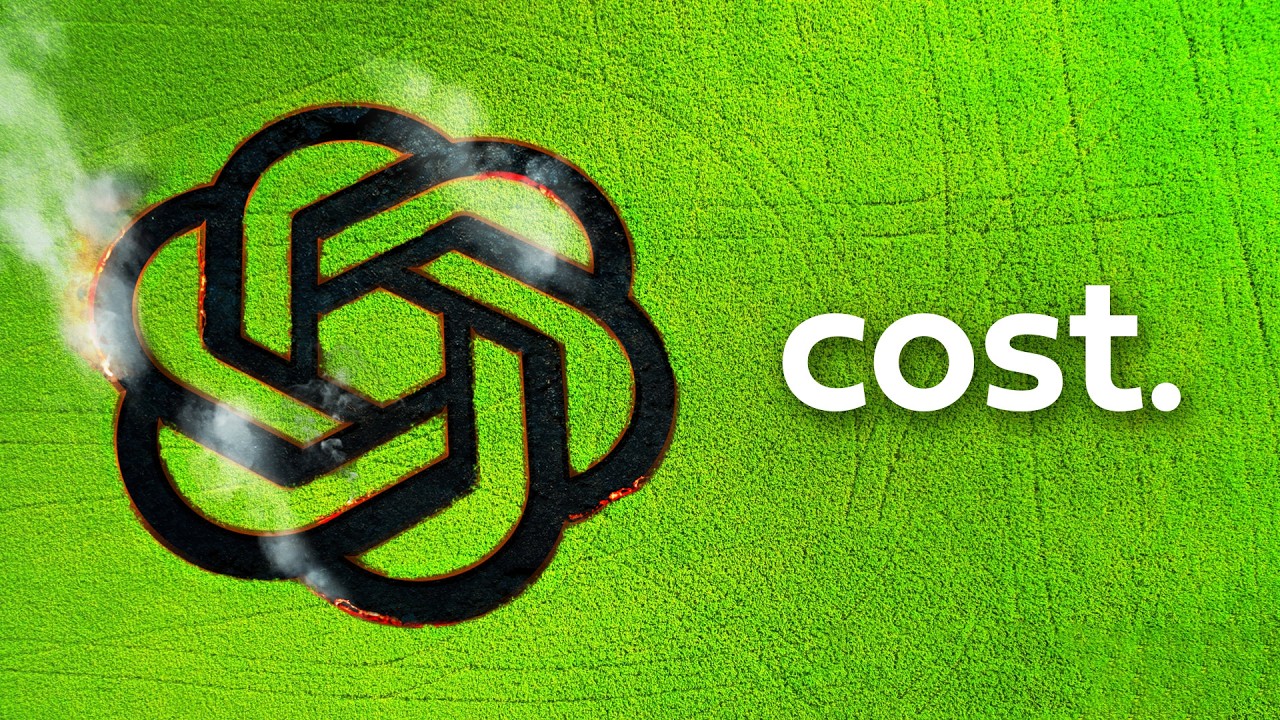
Create articles from any YouTube video or use our API to get YouTube transcriptions
Start for freeThe Energy Footprint of AI
Artificial intelligence (AI) has become an integral part of our daily lives, but its environmental impact is a growing concern. Let's break down the energy consumption of some common AI tasks:
- Asking a question to a large language model like ChatGPT uses approximately 3.6 joules of energy, equivalent to powering an LED bulb for 1 second.
- Using an AI tool to convert speech to text consumes about 79 joules.
- Generating an image from a text prompt requires around 1,700 joules, enough to power a laptop for about 30 seconds.
With ChatGPT alone processing over 1 billion queries each day, it's clear that AI tools are consuming significant amounts of energy. This rapid growth in AI usage has sparked debates about its potential environmental damage.
The Life Cycle of AI Technologies
To fully understand the environmental impact of AI, we need to examine its entire life cycle:
Hardware Production
AI requires specialized hardware with fast parallel processing capabilities. This involves the use of specific minerals like gallium and germanium, as well as ultra-pure water. The environmental costs associated with these materials are significant:
- Gallium extraction, primarily done in China (98% of low-purity gallium production), produces substantial waste and pollution in air, water, and soil.
- The semiconductor industry uses an estimated 1.2 billion cubic meters of water annually.
While this water usage is significant, it's important to note that it's still less than 0.1% of what agriculture uses yearly.
Model Training
Training AI models has historically been the most energy-intensive part of the process. It involves running complex algorithms on vast datasets, which often include:
- Transcripts of YouTube videos
- Text from entire books
- Other large-scale data sources
The ethical concerns surrounding data collection for AI training are significant and deserve their own discussion.
The energy consumption during training is substantial but difficult to quantify precisely. Companies are often not transparent about their energy usage. Additionally, water is used to cool the hardware during this process. For example, training the GPT-3 model evaporated 700,000 liters of clean fresh water.
Model Usage
As AI tools become more complex and popular, the energy consumed during usage is now outpacing that of training. For models like newer versions of ChatGPT, usage energy surpasses training energy within 2-3 weeks of public release.
This energy is primarily consumed in data centers, not on end-user devices. The concentration of these data centers in specific areas puts significant strain on local energy grids:
- In Ireland, data centers account for 17% of all electricity use.
- In more than five U.S. states, data centers consume over 10% of electricity demand.
Hardware Disposal
Eventually, the hardware used in AI systems will need to be recycled or disposed of. Currently, only about 20% of computer hardware is recycled, leading to potential environmental hazards from e-waste.
The Scale of AI's Environmental Impact
The rapid growth of AI usage is causing significant increases in energy consumption:
- Google's carbon emissions in 2023 were 48% higher than in 2019, partly due to increased AI service usage.
- Many tech giants are revising or abandoning their previous carbon reduction commitments.
Future projections vary widely:
- The International Energy Agency estimates that by 2030, data centers could require an additional 118-383 terawatt-hours of electricity per year.
- However, these predictions are often revised as hardware efficiency improves and new, more efficient models are developed.
Potential Environmental Benefits of AI
Despite its energy consumption, AI also offers potential environmental benefits:
- AI-driven atmospheric models can produce weather forecasts comparable to supercomputer-generated ones but using far less energy.
- Google aims to use AI to improve efficiencies in transport networks, power systems, and logistics, potentially avoiding 1 billion tons of carbon emissions annually by 2030.
- AI can help build smart grids, improve energy efficiency, and reduce fertilizer use in agriculture.
- AI chatbots have shown effectiveness in challenging climate skeptics' beliefs.
However, these benefits come with caveats. For instance, AI models trained on historical data may struggle to predict weather accurately in a changing climate, and efficiency improvements could potentially be applied to carbon-intensive industries as well.
Individual Use and Environmental Impact
For most individual users, the environmental impact of AI tool usage is relatively small compared to other daily activities. A study comparing the carbon intensity of AI-generated text and images to human-created content found that AI was significantly more carbon-efficient.
However, this doesn't mean AI use is without environmental cost. The key question is whether AI tools are replacing human effort or enabling increased productivity and consumption.
Broader Implications and Ethical Considerations
The environmental impact of AI cannot be separated from its broader socioeconomic effects:
- Data used to train AI models often raises ethical concerns about intellectual property and consent.
- The rapid development of AI tools by large tech companies is driven by the promise of future profits, often at the expense of immediate environmental considerations.
- The lack of regulation in the AI industry has allowed companies to prioritize growth over environmental and ethical concerns.
The Path Forward
Addressing the environmental impact of AI requires a multi-faceted approach:
-
Increased Transparency: AI companies should be required to disclose the full environmental impact of their products.
-
Regulation: Governments need to implement and enforce regulations that balance innovation with environmental protection.
-
Collective Action: Instead of focusing solely on individual choices, we need organized movements to push for systemic changes in how AI is developed and deployed.
-
Comprehensive Cost Calculation: The environmental costs of AI should be factored into development decisions, rather than being treated as externalities to be solved by future technologies.
-
Sustainable AI Development: Encourage research and development of more energy-efficient AI models and hardware.
-
Renewable Energy Integration: Push for data centers and AI operations to be powered by renewable energy sources.
-
Ethical Data Practices: Implement stricter guidelines on data collection and usage for AI training.
-
Public Awareness: Educate the public about the environmental implications of AI to foster informed decision-making and support for sustainable AI practices.
Conclusion
The environmental impact of AI is complex and multifaceted. While individual usage may have a relatively small footprint, the cumulative effect of widespread AI adoption poses significant challenges. However, AI also offers potential solutions to environmental problems.
The key lies in balancing innovation with sustainability. This requires a collective effort from tech companies, policymakers, researchers, and users. By approaching AI development and deployment with environmental consciousness, we can harness its benefits while mitigating its negative impacts.
As we continue to integrate AI into various aspects of our lives, it's crucial to remain vigilant about its environmental consequences. Through informed decision-making, responsible development, and collective action, we can work towards a future where AI serves as a tool for environmental progress rather than a contributor to environmental degradation.
Article created from: https://youtu.be/5sFBySzNIX0?si=Dl7YWGToepvAL6_g