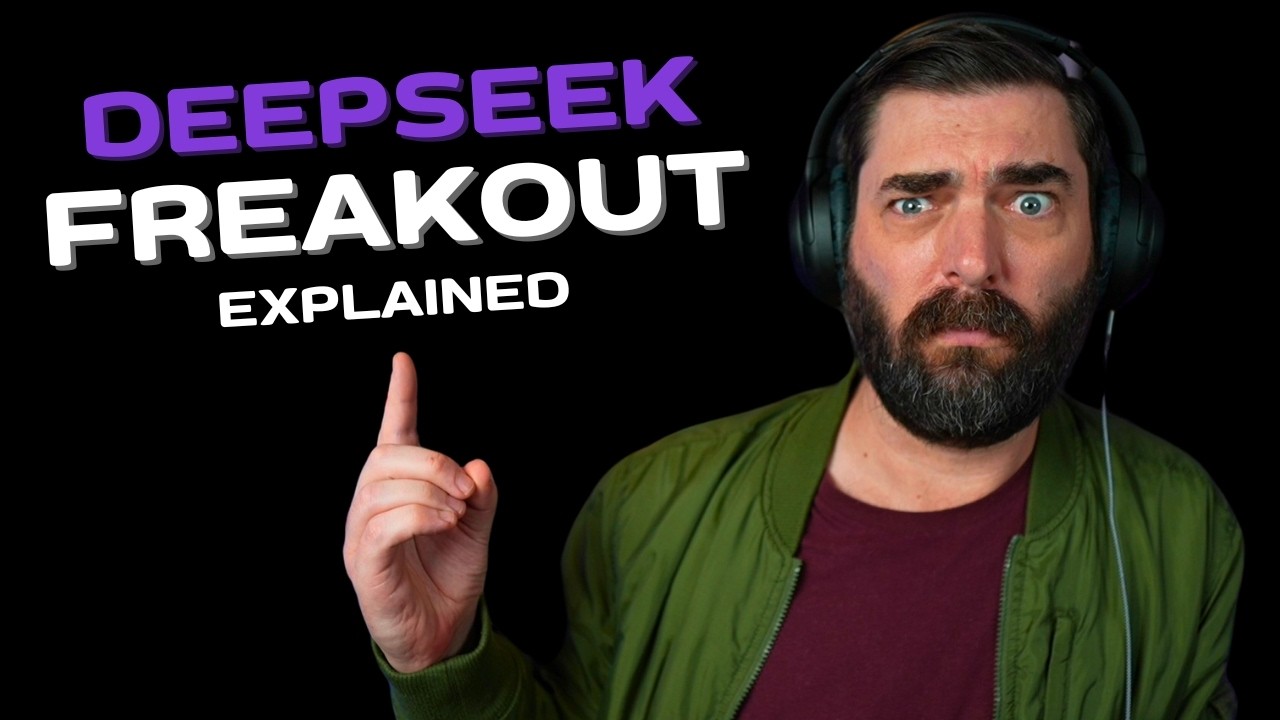
Create articles from any YouTube video or use our API to get YouTube transcriptions
Start for freeThe AI Advancement Causing a Stir
A new AI breakthrough called Deep Seek has recently emerged, causing significant ripples across the tech industry. On January 27, 2025, Nvidia experienced a substantial stock drop, losing over 17.7% of its value - equivalent to $465 billion in market capitalization. This dramatic shift has been attributed to the release of Deep Seek, an open-source AI model that's challenging the status quo.
Andreessen Horowitz, a prominent Silicon Valley investor, described Deep Seek R1 as "one of the most amazing and impressive breakthroughs I've ever seen" and praised it as "a profound gift to the world." But what exactly is Deep Seek, and why is it causing such a commotion in the AI community?
Understanding Deep Seek V3
To comprehend the significance of Deep Seek R1, we need to first examine its predecessor, Deep Seek V3. This large language model, introduced in December 2024, boasted 671 billion parameters. However, it utilized a "mixture of experts" approach, meaning it didn't use all parameters for every prompt. Instead, it activated only 37 billion parameters per token.
What made Deep Seek V3 truly remarkable was its efficiency. The model required only 2.78 million H800 GPU hours for its full training. To put this into perspective, GPT-4's training reportedly required approximately 60 million GPU hours. This means Deep Seek V3 was trained about 95% faster than GPT-4, and on less powerful hardware.
Moreover, Deep Seek V3 achieved impressive benchmark results:
- In mathematics, it outperformed GPT-4 and scored similarly to Claude 3.5.
- On the MMLU (which tests various tasks for large language models), it ranked second, only behind Claude 3.5.
- In coding benchmarks, it significantly outperformed other models.
- On SWE Bench (testing AI performance on GitHub problems), it was only slightly outscored by Claude 3.5.
The Leap to Deep Seek R1
While Deep Seek V3 was impressive, the real game-changer came with the introduction of Deep Seek R1. This new model uses Deep Seek V3 as its foundation but incorporates additional innovations:
-
New Fine-Tuning Method: R1 underwent a novel fine-tuning process using large-scale reinforcement learning without supervised fine-tuning.
-
Chain of Thought Prompting: When given a prompt, R1 demonstrates its reasoning process, sometimes even correcting itself, providing insight into its decision-making.
-
Improved Performance: R1 has shown results on par with or better than closed-source models like OpenAI's GPT-4.
Benchmarks and Comparisons
Deep Seek R1's performance has been turning heads in the AI community. When compared to other leading models:
- It outperformed or matched OpenAI's GPT-4 in most benchmarks.
- For coding tasks, it surpassed GPT-4.
- In mathematics, it led the pack.
- For general-purpose use, it performed comparably to GPT-4.
- In solving GitHub problems, it emerged as the leader.
What makes these results particularly striking is that Deep Seek R1 achieved this level of performance with significantly less computational resources and training time compared to its competitors.
The Impact on the Tech Industry
The emergence of Deep Seek R1 has sent shockwaves through the tech industry, particularly affecting companies heavily invested in AI hardware and development:
-
Stock Market Reaction: Nvidia, a leading manufacturer of GPUs used in AI training, saw a significant stock drop. This ripple effect also impacted other tech giants like Meta, Google, and Oracle.
-
Questioning the Need for Expensive Hardware: The efficiency of Deep Seek R1 has led some to question whether the massive amounts of high-end GPUs currently used in AI training are necessary.
-
Democratization of AI: As an open-source model achieving results comparable to closed-source alternatives, Deep Seek R1 could potentially democratize access to cutting-edge AI capabilities.
Controversies and Speculations
Despite the excitement surrounding Deep Seek R1, some controversies and speculations have emerged:
-
Hardware Used: Some analysts, including those at Citibank, have expressed doubt about whether Deep Seek truly achieved its results using less advanced chips. There's speculation that more powerful GPUs might have been used but not disclosed due to export controls.
-
Starting Point: Rumors suggest that Deep Seek might not have started from scratch but instead built upon existing models like LLaMA. However, there's little concrete evidence to support these claims.
-
Accuracy of Reported Resources: There's ongoing debate about the accuracy of Deep Seek's claims regarding the number and type of GPUs used in training.
Potential Long-Term Implications
While the immediate reaction to Deep Seek R1 has been a market shift, the long-term implications could be more nuanced:
-
Increased Efficiency Might Drive More Demand: If AI model training becomes more efficient, it could lower the barrier to entry for more companies to develop their own models, potentially increasing overall demand for computational resources.
-
Push for Even More Powerful Models: With the knowledge that powerful models can be trained more efficiently, companies might invest in even more extensive training to push the boundaries further.
-
Acceleration of AI Adoption: As AI becomes more accessible and efficient, its use in real-world applications could grow exponentially, driving demand for inference capabilities.
How to Use Deep Seek
For those interested in experiencing Deep Seek firsthand, there are several ways to access it:
-
Deep Seek Website: Visit deep seek.com and use the model directly through their web interface.
-
Mobile App: Deep Seek is available as a mobile app, currently ranking as the top free app in some app stores.
-
Grock Console: A distilled version of Deep Seek is available through console.gro.com, using a LLaMA 70B model with R1's reasoning capabilities.
-
Local Installation: Tools like LM Studio allow users to run distilled versions of Deep Seek locally on their own computers, ensuring privacy and offline access.
Beyond Language Models: Janice Pro 7B
In a surprising development, the same company behind Deep Seek has also introduced Janice Pro 7B, an AI image generation model. Early benchmarks suggest that Janice Pro 7B outperforms several leading image generation models, including SDXL, Stable Diffusion 1.5, DALL-E 3, and others.
This expansion into image generation demonstrates the company's ambition to disrupt multiple areas of AI technology, not just language models.
Conclusion
Deep Seek R1 represents a significant milestone in AI development, showcasing the potential for more efficient and accessible AI models. Its emergence has sparked discussions about the future of AI hardware, the democratization of AI technology, and the potential for rapid advancements in the field.
While the immediate market reaction has been volatile, the long-term implications of this technology are yet to be fully understood. It's clear, however, that Deep Seek has accelerated the pace of innovation in AI and challenged existing paradigms about computational requirements for advanced AI models.
As the AI landscape continues to evolve, it will be crucial to monitor how established players respond to these developments and how the broader tech industry adapts to the potential for more efficient and powerful AI models. Whether Deep Seek R1 marks the beginning of a new era in AI development or serves as a catalyst for further innovations from other companies remains to be seen.
For now, the availability of Deep Seek R1 as an open-source model provides an opportunity for researchers, developers, and enthusiasts to explore its capabilities and potentially build upon its foundations. As we move forward, the impact of Deep Seek on both the technical and economic aspects of AI development will undoubtedly be a topic of continued discussion and analysis in the tech world.
Article created from: https://youtu.be/9TU2Ootf7QE?feature=shared