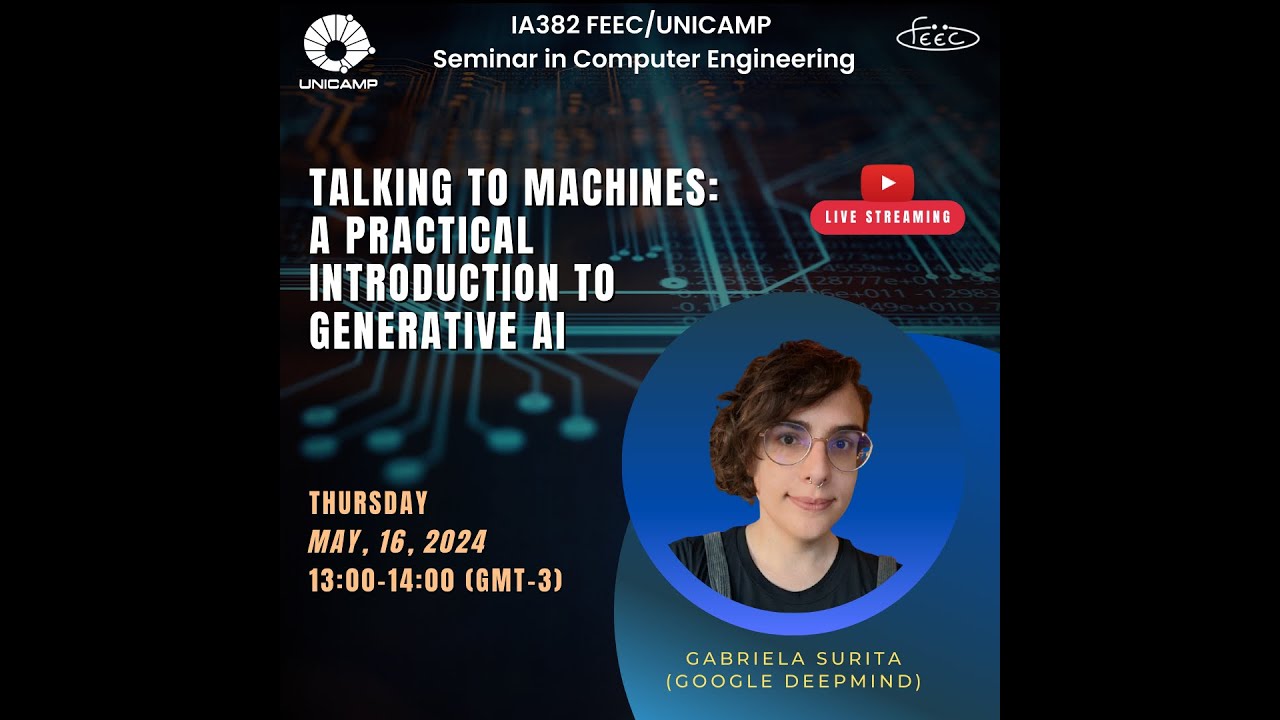
Create articles from any YouTube video or use our API to get YouTube transcriptions
Start for freeIntroduction to Generative AI
Generative AI stands out in the tech world for its ability to create human-like digital content such as text, images, and audio. Unlike predictive models that focus on statistical tasks, generative AI thrives in creative, open-ended environments. Gabriela Surita from Google DeepMind recently shared insights on this technology during a seminar at Unicamp.
Understanding Sequence Modeling
A core component of generative AI is sequence modeling. This technique predicts the next element in a sequence based on previous elements. For instance, predicting the next word in a sentence or the next note in a melody. This capability is fundamental for applications like chatbots or advanced text editors.
The Role of Neural Networks
Neural networks play a crucial role by approximating large sets of data with dense vectors, allowing for efficient handling of complex sequences. Recent advancements have led to more powerful models like Transformers, which significantly enhance the training and application of these networks.
Practical Applications and Tips
Surita emphasized practical strategies for leveraging generative AI effectively:
- Formatting and Tokenization: Essential for converting natural language input to a form that models can process efficiently.
- Demonstration-Based Learning: Providing examples can significantly improve model performance compared to abstract descriptions alone.
- Avoid Building from Scratch: Utilizing pre-built models from reputable sources can save time and resources while ensuring robust performance.
- Bias Mitigation: Instruction tuning can help address biases inherent in training data sourced from the internet.
Philosophical Considerations
Generative AI also brings up philosophical questions about technology's role and impact:
- Emergentism: The belief that complex systems cannot be fully understood by analyzing their components separately.
- Anthropomorphism: The tendency to attribute human characteristics to non-human entities, which can lead to unrealistic expectations about what AI can do.
- Shannon's Theory: Highlights how meaning is detached from data in communication processes, challenging us to think about how we interpret outputs from AI systems.
Ethical Implications and Future Directions
The ethical dimensions of generative AI are profound. Ensuring fairness and avoiding discrimination are critical challenges as these technologies become more integrated with daily life. Surita advocates for diverse testing teams and continuous monitoring to mitigate potential biases effectively.
In conclusion, while generative AI offers significant opportunities for innovation, it also requires careful consideration of both technical capabilities and broader societal impacts. By understanding both the mechanisms behind these tools and their implications, we can better harness their potential responsibly.
Article created from: https://www.youtube.com/watch?v=SOg-SOl2lO0