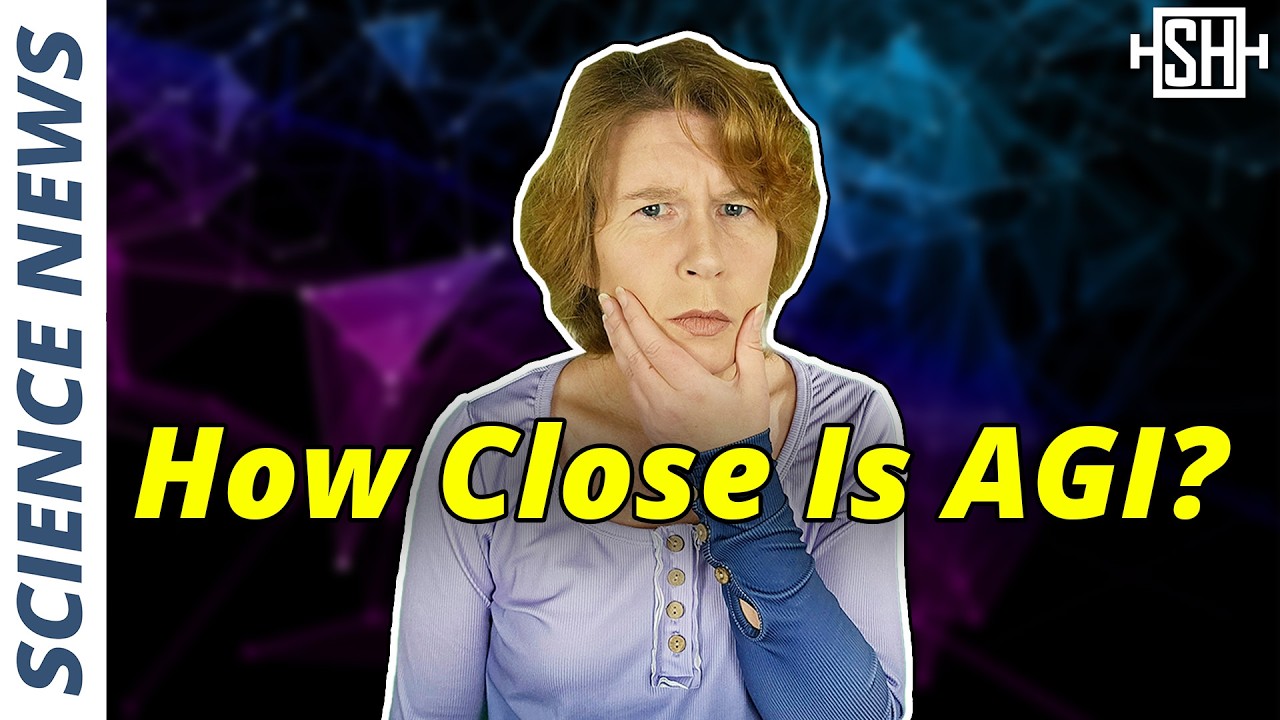
Create articles from any YouTube video or use our API to get YouTube transcriptions
Start for freeThe Rise of GPT-3.5 and Its Implications
In the rapidly evolving field of artificial intelligence, the end of last year saw a significant development with OpenAI's announcement of their newest model, GPT-3.5. This release sparked considerable discussion and speculation about the proximity of machines achieving human-level intelligence. Let's examine the capabilities of GPT-3.5 and what they might mean for the future of AI.
GPT-3.5's Impressive Test Scores
GPT-3.5 has shown remarkable performance in several key areas:
-
EPO Frontier Math: This test consists of complex mathematical problems that would challenge even highly skilled mathematicians. While previous AI models managed to solve only 2% of these problems correctly, GPT-3.5 achieved an impressive 25% success rate.
-
Code Generation: GPT-3.5 can produce code faster and with less computational power than its predecessors. This translates to an order of magnitude improvement in speed and cost-effectiveness for the same or better code performance.
-
ARC-AGI Test: The Abstract and Reasoning Corpus for Artificial General Intelligence (ARC-AGI) test consists of graphical puzzles designed to assess reasoning capabilities. GPT-3.5 scored 75% at low compute and 87% at high compute, surpassing the previous record of 55%.
The Cost Factor
It's worth noting that these impressive results come at a significant cost. The high-compute version of GPT-3.5 can cost up to $33,000 for a single task, compared to a few dollars for previous models. This raises questions about the scalability and accessibility of such advanced AI systems.
Comparing AI to Human Intelligence
The average human score on the ARC-AGI test is approximately 76%, which is comparable to GPT-3.5's performance. This has led some to speculate about the proximity of Artificial General Intelligence (AGI). Steven Heyle from OpenAI even commented on social media that "it's beginning to look a lot like AGI."
However, it's crucial to understand that passing this test doesn't necessarily indicate the achievement of AGI. Rather, it suggests that if an AGI were to exist, it should be able to pass this test.
The Challenge of Defining AGI
One of the main obstacles in discussing AGI is the lack of a universally accepted definition. Even Sam Altman, the CEO of OpenAI, has expressed discomfort with the term. Despite this, he has made bold predictions about AI capabilities:
"By the end of 2025, I expect we will have systems that can do truly astonishing cognitive tasks, where you'll use it and be like, 'Wow, that thing is smarter than me at a lot of hard problems.'" - Sam Altman
The Scaling Hypothesis and Its Limitations
Many in the AI industry believe in the scaling hypothesis - the idea that large language models will continue to improve as they are fed more data, eventually surpassing human intelligence. This hypothesis has been observed in many previous models.
However, recent indications suggest that progress on large language models (LLMs) may be slowing down. Some experts, like Yann LeCun from Meta, believe that LLMs are not the path to AGI and that true AGI won't arrive before the end of the decade.
The Limitations of Current AI Models
Despite their impressive performance on specific tasks, current AI models still fall short in many areas of general intelligence:
- They can't learn to drive in 20 hours like a typical 17-year-old.
- They struggle with simple tasks that a 10-year-old can learn quickly, such as clearing a dinner table and loading a dishwasher.
- They lack the ability to generalize learning from one domain to another, a key aspect of human intelligence.
Expert Opinions on AGI Timeline
Experts in the field have varying opinions on when we might achieve AGI:
Yann LeCun (Meta)
LeCun believes that AGI is not imminent:
"It's not going to happen next year... What may happen in the next two years is that it's going to be more and more difficult to find cases where common people will be able to ask questions to the latest chatbot that the chat would not be able to answer."
Gary Marcus (Cognitive Scientist)
Marcus argues that AGI is much harder to achieve than many in the field currently believe:
"AI is harder than its originators realized, and actually, it's harder than most of the people who are hyping the field right now realize, which includes CEOs of companies like OpenAI."
He also criticizes the current focus on transformer models:
"We have an intellectual monoculture in which almost all of the research dollars and energy goes towards Transformer models and almost nothing else, and that's insane."
The Future of AI Research and Development
Given the enormous investments made in training current AI models, it's unlikely that companies like OpenAI and Anthropic will completely abandon their current approaches. Instead, we might see a shift in focus:
-
Niche Applications: Companies may concentrate on finding specific areas where their models perform well enough to be profitable.
-
Redefining AGI: There are indications that some companies might redefine AGI in terms of profitability rather than human-like intelligence. For instance, internal documents between Microsoft and OpenAI reportedly define AGI as any system that will make more than $100 billion in profit.
-
Monetization Strategies: Companies may explore various ways to monetize their AI models, including potentially incorporating advertisements.
The Need for Diverse Approaches in AI Research
The current focus on transformer models and large language models may be limiting progress towards true AGI. To overcome this, the AI research community should consider:
-
Diversifying Research Approaches: Exploring alternative architectures and learning paradigms beyond transformer models.
-
Interdisciplinary Collaboration: Incorporating insights from cognitive science, neuroscience, and other related fields to inform AI development.
-
Addressing Fundamental Challenges: Focusing on core issues such as common-sense reasoning, causal understanding, and transfer learning.
Ethical Considerations and Safety Concerns
As AI systems become more advanced, it's crucial to address the ethical implications and potential risks:
Data Privacy and Security
The vast amounts of data required to train advanced AI models raise concerns about privacy and data security. It's essential to develop robust frameworks for data protection and ethical data usage.
AI Safety
Ensuring the safety and reliability of AI systems becomes increasingly important as they are integrated into critical applications. This includes addressing issues such as:
- Algorithmic bias
- Robustness to adversarial attacks
- Alignment with human values
Societal Impact
The potential impact of advanced AI on employment, social structures, and decision-making processes needs careful consideration and proactive planning.
The Role of Regulation in AI Development
As AI technology advances, the need for appropriate regulation becomes more pressing. Key areas for regulatory focus include:
-
Transparency: Requiring AI companies to be more transparent about their models' capabilities, limitations, and potential risks.
-
Accountability: Establishing clear lines of responsibility for AI-driven decisions and actions.
-
Ethical Guidelines: Developing and enforcing ethical standards for AI development and deployment.
-
International Cooperation: Fostering global collaboration to address the challenges and opportunities presented by AI.
The Importance of Public Understanding and Engagement
As AI continues to shape our world, it's crucial to promote public understanding and engagement with these technologies:
-
AI Literacy: Developing educational programs to improve public understanding of AI capabilities and limitations.
-
Public Discourse: Encouraging open discussions about the societal implications of AI advancements.
-
Participatory Decision-making: Involving diverse stakeholders in shaping AI policies and development priorities.
Conclusion: The Path Forward
While recent advancements in AI, such as GPT-3.5, are impressive, we are still far from achieving true Artificial General Intelligence. The path to AGI is likely to be longer and more complex than some optimistic predictions suggest.
Moving forward, it's essential to:
- Maintain a balanced perspective on AI capabilities and limitations.
- Encourage diverse approaches in AI research and development.
- Address ethical concerns and safety issues proactively.
- Develop appropriate regulatory frameworks.
- Foster public understanding and engagement with AI technologies.
By taking a thoughtful and multifaceted approach to AI development, we can work towards realizing the potential benefits of these technologies while mitigating potential risks and challenges. The journey towards AGI may be long, but it promises to be one of the most transformative endeavors in human history.
Article created from: https://www.youtube.com/watch?v=pz9FQ1gwh3g