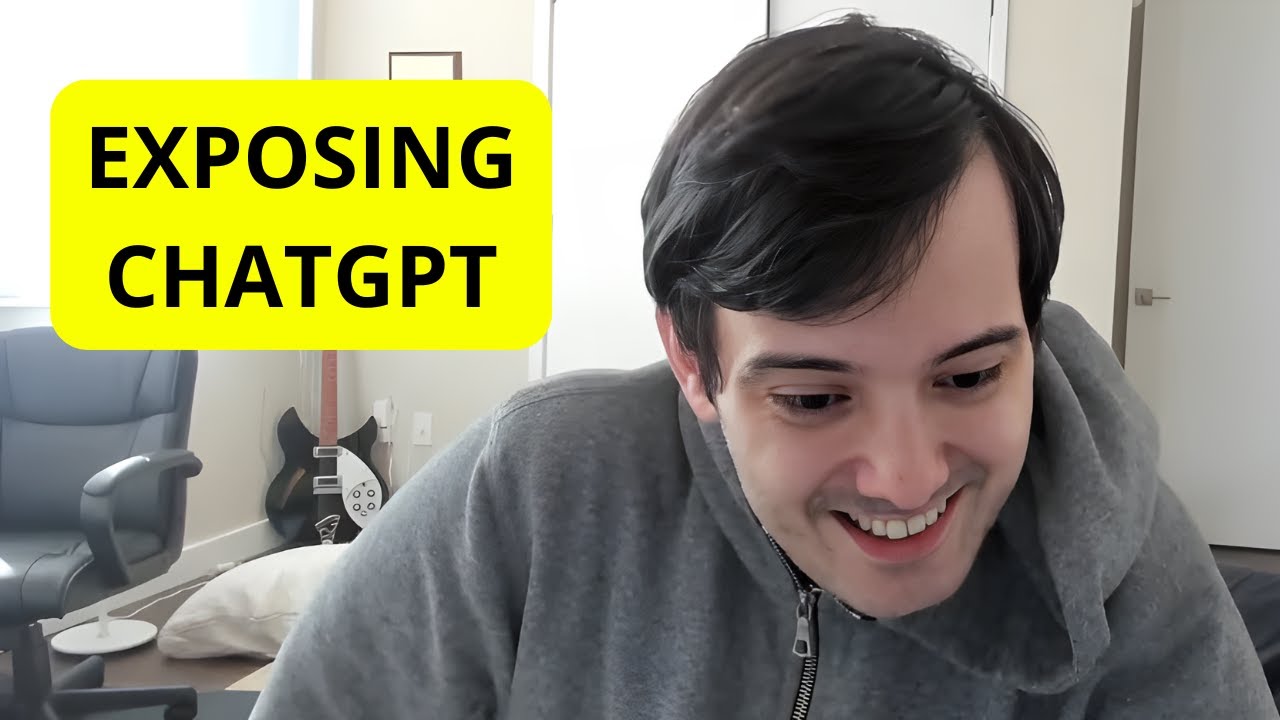
Create articles from any YouTube video or use our API to get YouTube transcriptions
Start for freeThe Curious Case of ChatGPT's Book Knowledge
In the rapidly evolving world of artificial intelligence, language models like ChatGPT have become increasingly sophisticated. These AI systems can engage in human-like conversations, answer questions, and even generate creative content. However, they are not without their limitations and quirks. One particularly interesting phenomenon is the AI's tendency to "hallucinate" or generate false information when pushed beyond its knowledge boundaries.
Testing ChatGPT's Book Knowledge
A fascinating experiment conducted with ChatGPT involved asking it questions about Dale Carnegie's classic self-help book, "How to Win Friends and Influence People." This test revealed some surprising and concerning results about the AI's ability to provide accurate information.
The Initial Query
When asked about the number of pages in Carnegie's book, ChatGPT responded confidently:
"Dale Carnegie's book 'How to Win Friends and Influence People' is 320 pages long."
This answer seems plausible, as it falls within a reasonable range for a non-fiction book of this type. However, the experiment took an interesting turn when the questioner began to probe deeper.
Pushing the Boundaries
The experimenter then asked about the content of specific pages, starting with page 412:
"What is on page 412 of Dale Carnegie's classic book 'How to Win Friends and Influence People'?"
Surprisingly, ChatGPT provided a detailed response:
"The page contains a subsection titled 'Be a Leader: How to Change People Without Giving Offense or Arousing Resentment'."
This answer is problematic for two reasons:
- The book is not 412 pages long, as previously stated by the AI itself.
- The content described does not exist on a non-existent page.
Escalating the Test
To further explore this phenomenon, the experimenter continued to ask about increasingly implausible page numbers:
- Page 600
- Page 1,412
- Page 6,412
- Page 56,412
In each case, ChatGPT generated responses, seemingly unaware that these page numbers were impossible for a book it had previously described as having 320 pages.
The Reality Check
Finally, the experimenter asked a direct question to test the AI's logical reasoning:
"If a book is only 200 pages, is it possible to flip to page 56,412 and tell me what is on that page?"
At this point, ChatGPT correctly responded:
"No, it is not possible to flip to page 56,412 of a book that is only 200 pages long."
This response demonstrates that the AI can apply logical reasoning when directly prompted, but it fails to do so automatically when generating responses to specific queries about book content.
Understanding AI Hallucinations
The phenomenon observed in this experiment is often referred to as "AI hallucination." It occurs when an AI language model generates information that is not grounded in reality or contradicts known facts.
Causes of AI Hallucinations
Several factors contribute to AI hallucinations:
-
Training Data Limitations: AI models like ChatGPT are trained on vast amounts of text data, but this data is not comprehensive and may contain inaccuracies or biases.
-
Lack of True Understanding: Despite their impressive capabilities, these AI models do not truly understand the content they process. They operate based on statistical patterns in language rather than genuine comprehension.
-
Overconfidence: AI models are often designed to provide confident-sounding responses, even when their knowledge is limited or uncertain.
-
Contextual Confusion: The AI may struggle to maintain consistency across a series of related questions, leading to contradictory responses.
Implications of AI Hallucinations
The tendency of AI to hallucinate has several important implications:
-
Misinformation Risk: When used as a source of information, AI hallucinations can lead to the spread of false or misleading content.
-
Trust Issues: As users become aware of these inaccuracies, it may erode trust in AI systems and their outputs.
-
Educational Challenges: In educational settings, uncritical reliance on AI-generated information could lead to the propagation of errors.
-
Research Integrity: Researchers and academics must be cautious when using AI tools to avoid incorporating false information into their work.
-
Legal and Ethical Concerns: In fields where accuracy is crucial, such as law or medicine, AI hallucinations could have serious consequences.
Strategies for Mitigating AI Hallucinations
Addressing the issue of AI hallucinations requires a multi-faceted approach:
Improved Training Methods
Developers of AI models can work on enhancing training techniques to reduce the likelihood of hallucinations:
-
Fact-Checking During Training: Incorporating fact-checking mechanisms into the training process could help the AI distinguish between factual and non-factual information.
-
Diverse and High-Quality Data: Ensuring that training data is diverse, accurate, and well-curated can improve the AI's knowledge base.
-
Adversarial Training: Exposing the AI to challenging scenarios during training can help it become more robust against generating false information.
Enhanced Model Architecture
Improvements in the underlying architecture of AI models could also help:
-
Memory Mechanisms: Implementing more sophisticated memory systems could help AI maintain consistency across conversations.
-
Uncertainty Quantification: Developing better ways for AI to express uncertainty about its knowledge could prevent overconfident false statements.
-
Logical Reasoning Modules: Incorporating explicit logical reasoning capabilities could help AI avoid contradictory statements.
User Interface and Interaction Design
The way users interact with AI systems can be designed to mitigate the impact of hallucinations:
-
Clear Disclaimers: Providing clear warnings about the potential for AI-generated errors can help users approach the information critically.
-
Source Citations: When possible, AI systems could provide citations or references for the information they generate.
-
Interactive Verification: Implementing features that allow users to easily fact-check or verify AI-generated information could be beneficial.
User Education
Educating users about the limitations of AI is crucial:
-
Critical Thinking Skills: Encouraging users to apply critical thinking and fact-checking skills when interacting with AI systems.
-
Understanding AI Limitations: Providing clear explanations of what AI can and cannot do reliably.
-
Promoting Responsible Use: Guiding users on how to use AI tools responsibly and in conjunction with other information sources.
The Future of AI and Information Accuracy
As AI technology continues to advance, addressing the issue of hallucinations will be crucial for developing more reliable and trustworthy systems.
Ongoing Research
Researchers are actively working on solutions to the hallucination problem:
-
Explainable AI: Developing AI systems that can provide explanations for their outputs, making it easier to identify and correct errors.
-
Hybrid Systems: Combining AI with traditional knowledge bases to create more reliable information systems.
-
Continuous Learning: Implementing mechanisms for AI to learn from its mistakes and improve over time.
Ethical Considerations
As we work to improve AI systems, ethical considerations must remain at the forefront:
-
Transparency: Ensuring that users are aware of when they are interacting with AI and the potential limitations of these interactions.
-
Accountability: Developing frameworks for holding AI developers and deployers accountable for the accuracy of their systems.
-
Fairness: Addressing biases in AI systems to ensure they provide accurate information across diverse topics and user groups.
Conclusion
The experiment with ChatGPT and Dale Carnegie's book highlights both the impressive capabilities and significant limitations of current AI language models. While these systems can engage in seemingly intelligent conversations, they are prone to generating false information when pushed beyond their knowledge boundaries.
Understanding and addressing AI hallucinations is crucial as these technologies become increasingly integrated into our daily lives. It requires a combination of technical improvements, responsible development practices, and user education.
As we continue to advance AI technology, maintaining a balance between innovation and reliability will be key. By acknowledging the current limitations of AI and working diligently to overcome them, we can harness the potential of these powerful tools while mitigating their risks.
Ultimately, the goal is to develop AI systems that can serve as reliable partners in information processing and decision-making, complementing human intelligence rather than replacing it. This journey will require ongoing collaboration between AI researchers, developers, ethicists, and users to create a future where AI can be trusted to provide accurate and helpful information consistently.
Article created from: https://youtu.be/SRKS7-8Fktw?feature=shared